Review Article
Creative Commons, CC-BY
Computational Cybernetics of Glia-Neuron Cells Symbiosis versus Hopfield Recurrent Network: Do We Understand it? *
*Corresponding author: Georgi M Dimirovski is with Doctoral School FEIT of Saints Cyril & Methodius University in Skopje, 18 Rugjer Boskovic Str., MK-1000, North Macedonia.
Received: July 07, 2023; Published: July 19, 2023
DOI: 10.34297/AJBSR.2023.19.002608
Abstract
Science of artificial neural networks and relevant computing mechanisms since McCullock-Pitts artificial neuron [1] up via neurons and networks of Anderson (1972), Barto (1983), Grossberg (1967, 1976), Hopfield [2,3], Kohonen (1972) to Kasabov’s evolving connectionist systems with spiking-neurons [4] have undergone developments beyond any conceivable predictions. The computational efficiency and functionality of all kinds of neural network implies stable operating steady-state equilibrium is fast established and guaranteed. In parallel, Neurophysiology has yielded many insights Gayton-Hall [5] converging to paradigm of systems biology. It appeared, on the crossroad of these findings with Hilbert’s Thirteen problem and Kolmogorov’s Superposition Representations in conjunction with Lyapunov foundations of stability and LaSalle invariance principle certain delicate subtle issues emerged Siljak [6] and Sprecher [7]. This re-thinking the foundations of neural networks via the quest for parallels between artificial and living neurons is believed to open a new horizon. This belief follows obtained results on cultured-neuron controllers and recurrent neural networks with time-varying delays. A closer look into how animal and/or human brain cells can be cultivated as a controlling brain for a mobile robot (physical body) such that can move around and interact with the world. In turn, a new kind of artificial intelligence may be created, which is emulated by stabilized complex highly non-linear complex neural network system.
Introduction
The idea of intelligent self-organizing system [8] has been put forward long ago by W Ross Ashby hence undergone tremendous evolution since. On the other hand, during the last few years there is taking place an unprecedented rapid development of computing software [9,10] for various technological applications largely based on cognition science and pattern recognition [11,12]. Even more so claims have been put forward by various software applications all designated as the real renaissance of Artificial Intelligence (AI) developments [10,13]. Recently deceased genius Stephen W Hawking, in his 2014 arXive article on conservation of information and estimation of time for black holes, argued that we are facing the century of complexity in the scientific studies and its mathematical capturing that grounded on physics composed energy, matter and information. Also, Hawking warned on considerable danger from abuse of AI information based technologies, which is imminent if the respective underlying human background be neglected. The main issues seem to involve interaction, interference, and interplay of energy, matter and information within complex networks and systems [15-18] such as the human brain is [19-22].
It is therefore that the complexity of human brain network systemic structure and integrity of harmonized and integrated functioning is getting an open question the knowledge quest on which seems a never-ending kind of story. Moreover, Hawking’s warning emphasized how far reaching were the discoveries by David O Hebb (1949) on the learning organization of behaviour hence John J Hopfield’s [3,23] dynamic artificial neural networks, physically founded on sound electronic foundations with rigorous mathematical proves via Lyapunov stability theory. The core heart of each of these discoveries was built up around the idea of recurrent network structure of neurons in the functionality of which human cortex retains centrality role hence the human intelligence mind does so too. Nonetheless, computational functionality of the recurrent artificial neural networks implies the operating steadystate equilibrium is reached fast and first.
Recently, Forinto, et al., [24] have proposed a rather innovative treatise of the fundamentals of brain network analysis approach. It appeared, the main issues evolve among the interference, interaction, and interplay of energy, matter and information within the complex networks such as the human brain appears to be. It has been generally adopted to view the healthy human nervous system for each of its life-physiology functions as a specific three-stage dynamic system with a certain internal, but outer acting, feedback with capacity of receiving stimuli (external and internal ones) and responding by relevant reaction as appropriate. Cybernetic computational model of the unit of neuron inevitably remain crucial hence new hybrid models of spiking neurons and artificial neural networks of Kasabov [25,26] and Izhikevich [27,28], Izhikevich Edelman [29] possessing for reaching performance effects have emerged. In those remarkable discoveries, however, the discovery of life science on glia- neuron-cell co-existence [29-33] in mutually supportive symbiosis has not been observed before the initial study [34,35] by these authors. This paper reports on the new follow up findings established.
Further this paper is written as follows. The next section presents some of the background scientific foundations for the present follow up study. In the third section, there is presented first brief but relevant discussion to outline the ideas behind the proposed novel model of a neuronal unit in recurrent Hopfield network; thus it leads to an innovated representation model of Hopfield network as well. The concluding remarks along with points research and the references follow hereafter recently has been discovered. It should be noted that glia- cells are electrically non-excitable [36] despite they accompany every neuron. In turn, the ANN community does not pay attention to glia-neuron symbiosis [14,16,29,37] let alone it does the AI community at large. It is a biological fact, neurons– the primary functional units that are electrically excitable–do get into close and crucial morphological as well as functional symbiotic relationship with glia-cells. In turn these occupy almost half the volume of the compound tissue with neurons.
On Certain Background Foundations
The just published most comprehensive exploration study by Ed S. Lein, Gabor Tamas and co-authors [38] has proven that glianeuron- cell co-existence in symbiosis has not only vital but also far reaching on functionality of living neurons of human brain [39]. This fact has been discussed in SMC2016 paper by Dimirovski, et al., [19] at the same time argued it must be captured in the mathematical model of single artificial neuron (AN) in order to advance further computational power of artificial neural networks (ANN) along a road converging to living human neuronal network (LNN). This point appears rather crucial for the recurrent ANN aimed at mimicking human LNN.
Selected Points from Life Sciences
The life sciences based knowledge spectrum on recurrent neural networks delivered by Science of Neurophysiology [37] has yielded insights converging rather closer to the approach of Systems Biology Science [40]. Those considerably involved and non-transparent insights appear to fall on the crossroad of findings with Kolmogorov’s representation superposition and Hilbert’s Thirteen Problem hence yield emergence of certain rater delicate subtle issues as pointed out by Sprecher [7].
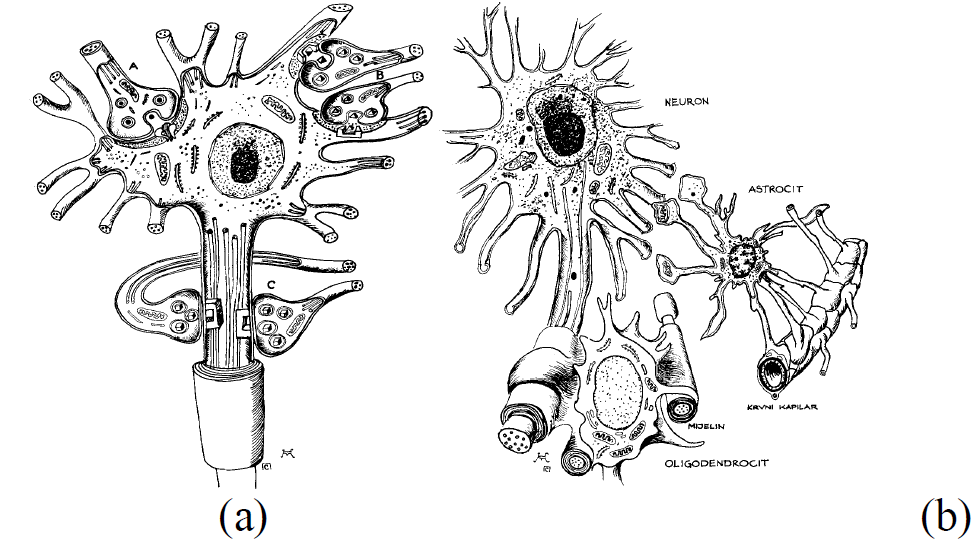
Figure 1: Schematic representation of living neuron physiology [22]: (a) on p.16, shoving synapses categories axon-somatic A, axon-dendritic B, and axon-axon C; and (b) on p. 26, showing symbiosis with glia-cell and blood vessels [19].
The present paper attempts to combine those two spectrumends in a cybernetic convolution by proposing an innovated electronic model of Hopfield recurrent ANNs based on the new model of the basic neuron unit employing the glia-neuron-cell symbiosis; see Figure 1. In particular the synaptogenesis in central neuronal system (CNS) [41,42] and the regulation of synaptic connectivity and communication [20,38,43] seem essentially dominated by this symbiosis. In [44], Buffo and Rossi [45] have shown clearly the origin lineage and function of cerebellar glia. Thus the closer examination neuron-glia symbiosis proposed in our SMC2017 paper [19] and investigation of its impact on revising the model of a single artificial neuron seems fully justified.
Physiological schematic of human neuron, as depicted in Figure 1, can be found in neuroscience literature where glia’s internal structure [20-22] is also involved. Though, the impact of glia on neuronal synapses [38,41,46] only recently has been discovered. It should be noted that glia cells are electrically non-excitable [21] despite they accompany every neuron. In turn, the ANN community does not pay attention to glia-neuron symbiosis [14,16,29,37] let alone it does the AI community at large. It is a biological fact, neurons–the primary functional units that are electrically excitable– do get into close and crucial morphological as well as functional symbiotic relationship with glia-cells. In turn these occupy almost half the volume of the compound tissue with neurons.
Complexity [47] of neuronal tissue organization exhibits remarkable features [19,34,48] much more than that in a circuit implementation of RANN [5,29]. It is at the very ‘heart’ of the basic neuron where neuron-cells and glia-cells are interconnected via numerous mountain-spoors dendrites, and where inter-cell space is astonishingly reduced to a– possibly systemic–set of too narrow channels (Figure 2).

Figure 2: Schematic of originally envisaged Hopfield networks based on Analogue Electronics (a) as depicted in [17]; Fig. 21-3 (2a) in there and a scan of its last segment (b); both global and local feedback loops are readily inferred emphasizing the recurrent dynamic features of Hopfield’s network system.
For these make a viable structure in which both cells accomplish their different as well as their specific functionality tasks. The comprehensive roles of this coupled complexity [10,19] is believed, to have been overlooked in more or less in all models of a neuron, in spite of its paramount importance understanding of the cybernetics of this complexity neuron- glia cells symbiosis appeals to revisit the modeling problem of the basic and primary unit of all neural networks.
For scientific reasons, first of all, we ought to refer to human central nervous system (CNS) where the neuron-glia symbiosis plays the crucial roles in human developmental life from the embryonic till the aging epochs [41]. It is not only for progressive daily functions and well-being. Though some of biochemical and molecular-biology mysteries of neuron- glia cells symbiosis only recently have been understood and uncovered; see works [4,10,11,20,21,29,30-32,36,49,50].
It is well known, interconnected neurons, via respective axondendrite synapses, constantly emit/receive chemically transmitted electrical signals thereby performing complex brain activities according to the processing of those signals, carrying biochemically encoded information Glia cells play crucial role in formation of synapses [44]. Furthermore, it was found, the glia-derived cholesterol is crucial for enhancing the CNS synapto-genesis [5], which largely governs most of mind functions and thus aspects of computational cybernetics [19]. As shown in [38], certain category of ions are transmitting signal to glia-cells, which likely then in turn enhance activities of the associated neuron-g ia cells. As shown in [45], there exists considerable ion-origin lineage enabling differentiation of glia-cells. It reflects on millennia natural evolution based, biological pattern recognition ‘computation’.
Brief Overview of Authors Previous Findings
Several recent investigation studies by these authors, albeit one departed from the stability problem of recurrent artificial neural network [19,35] while the other form creating complex biological controller of cultivated rat brain on appropriate electronic circuit [50-52], ultimately have led to the common conclusion that currently mathematical models of a basic neuron unit have neglected the symbiotic co-existence of glia-cell and neuron-cell within the neuronal body. On the other hand, the Hopfield recurrent artificial network [2,3,46] is well-known to mimic human brain recurrent neuronal system considerably better than any other neuromorphic electronic system (Maed, 1990) based artificial neural network. However, nonetheless the unit artificial neuron that has been used in so far still does not account for and observe the essential lifesupporting glia-neuron symbiosis [24,34].
Most recently these authors have embarked on exploration those findings and transcended them into novel ideas in ANN. There upon, they have made certain original innovation of the long existing computational neural networks such as multi-layer perceptron, involving models that capture glia’s impulse phenomena within neuron-glia cells interactions. In classical literature [37] and recent fundamental articles [20,30,38,40,44,45] the symbiotic coexistence of neuronal cells and the associated glia cells has been pointed out to be rather crucial. But only a few have studied deeply [21,31,36,49] in the context of the ANN. In Zhao, et al., [10] have observed the importance of neuron-glia symbiosis and have managed to develop a kind of extreme learning machine implementing soft computing decision-making for autonomous UAV missions.
Known Facts to Observe
A brief scanning of the selected references readily reveals the state of the art of both the feed-forward artificial neural networks (FANN) and the recurrent (RANN) ones [46,47,53]. The former have been well scientifically grounded via Kolmogorov’s functional superposition theory of functions (see Sprecher, 2017, for a comprehensive study). Yet, the same cannot be said for the latter albeit Hopfield and his followers [2,3,46,] as well as Kasabov and his school [25,26] have indeed achieved remarkable progresses in this direction.
Nonetheless these both represent supervisory-controlled selfstabilizing and feedback reference-tracking network, systems that may contain hidden thousands and millions local feedbacks just as the human brain. Thus, naturally more than just one unique asymptotically stable operating equilibrium state do exist. In conjunction with Izhikievich’s question [28] in his 2004 article (for full details of deeper scientific knowledge the references therein [28]) at this point. It is instrumental to revisit and carefully examine a brief, but illustrated, outline of what is pertinent to neuron [19]. These facts again support the innovated model as proposed in Figure 3 below.
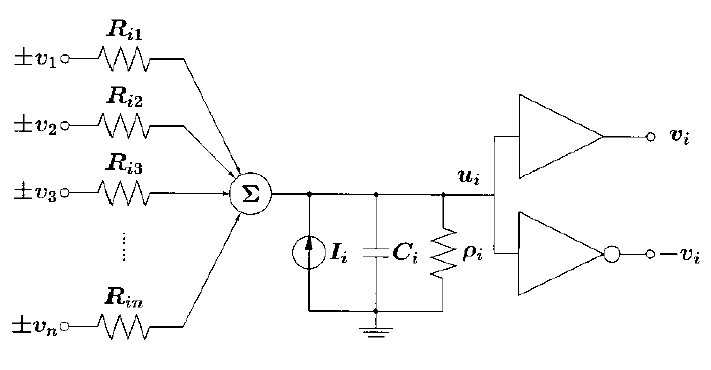
Figure 3: Novel representation model the neuron unit in Hopfield networks based on the investigations of these authors; in both cases the local feedback due to glia-neuron cell symbiosis is depicted by means of a varying resistor symbol in order to represent all the foreseen cases discussed above using either source models, of Khallil [54] or of Jang, et al. [55].
Guidance to Modify AN Model in Hopfield Networks
In order to present our understanding of neuron-glia symbiosis and its resulting impact on mutual functionality at neuronal level some facts on Hopfield networks [3,23] their outstanding computational cybernetics need to be recalled. In doing so, we shall make use of the Ch. 21 in the famous monograph [46] by Hagen and his collaborators; see Figure 2.
Figure 2A schematic of originally envisaged Hopfield networks based on Analogue Electronics (a) as depicted in [46]; Figure [3,45] (2a) in there and a scan of its last segment (b); both global and local feedback loops are readily inferred emphasizing the recurrent dynamic features of Hopfield’s network system.
It is well known in his pioneering research John J Hopfield [3,13] had used analogue electronic-electrical units employing amplifiers as perceived in 1980-ties. In his fundamental article [3] he had pointed out: “Any physical system whose dynamics in phase-space is dominated by a substantial number of locally stable states to which it is attracted can therefore be regarded as a general contentaddressable memory. The physical systems will be potentially useful memory if, in addition, any prescribed set of states can be readily made the stable set of the system.” This was the departure point in the previous studies [40,35] and [50-51] by these authors (Figure 3).
Remark 1: It should be noted, formally, the authors have used different schematic drawings of analogue electronic amplifier in Figures 2-5. We followed strictly Hopfield’s original conceptualization [23].
Thus, Khallil [54] has pointed out presence of both inverting and non-inverting outputs along with resistors and capacitors, as depicted in Figure 5. The pattern of chosen interconnections then allowed to derive the mathematical representation from Kirchhoff’s Current Law. This law elementary unit level obeys linear superposition but enhances nonlinear overall dynamics on the entire neural network due to possible potentially induced amplifier operating in saturation mode, i.e. the typically used sigmoid vi = f (neti ) [21,23].
Somewhat slightly modified schematic is found in Section 11.17 in Figure 11.15 of famous monograph by Jang, Sun and Mizutani [55] in order to emphasize the dynamics of Hopfield recurrent network; see Figure 4 below Focused attention to the glia-neuron cell symbiosis yields discovery at the neuron level local feedbacks shall improve considerably the overall operating capacity of this network by self-stabilizing operating equilibria [6,19,48] induced shown in [56,57]. Nonetheless, on the grounds of the biological cooperative neuron-glia co benefit notice a nonlinear-function based feedback is needed too. In turn the proposed novel model appears as in Figure 5 which depicts both variants by making of electronic schematics above: (a) innovated Khalil’s model in Figure 3; (b) innovated Jang’s model in Figure 4.
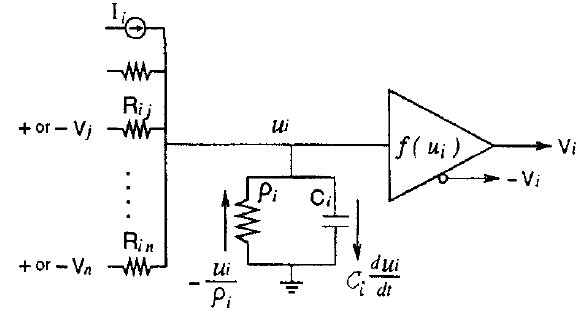
Figure 4: Schematic representation of the neuron unit in Hopfield networks based on physical electronics (a) as depicted in [55], Fig. 11.15 in Sec. 11.7.3.
Moreover, we believe, this nonlinear static feedback can be further extended by using self-stabilizing nonlinear systems in order to capture both the settled operating neuron-glia cells interactions and the switched ones between learning and nonlearning phases. It is therefore the innovated electronic schematic for artificial neurons proposed as depicted in Figure 3 below.
It is apparent now that writing the respective equations down, based on Kirchoff’s current law, is not that difficult task. Source equations of circuit in Figure 3 without feedback due to Khallil [54] are available in, while those of circuit in Figure 4 due Jang, et al., [55] are available in. In here, it is adopted to approximate mathematically the unit glia neuron using the circuit in Figure 3(a). Then, it remains in the next step only current term through the feedback resistor is to be included as well. Proportional feedback employing constant resistor RFBji is naturally the simplest and straightforward one. However, we have in mind various feasible feedback paths hence we use slightly modified symbols for variables and parameters:



are subject to design synthesis [34,40]; voltages are carrier of processed information signals. If output is taken from the inverting amplifies then signs would be opposite. It should be noted however, the issue of the varying resistor feedback is an open issue yet to be explored and design synthesis of function ( , ) FBji j N t v solved. Considerably many relevant variants may be conceived apparently, and one of those is to emulate adaptive control of switched nonlinear systems by Sun, et al., [47]. Of course, this is one of the future research endeavors by those interested to pursue further this line of exploration [35,50].
At this point it should be emphasized that the proposed innovated model has been found [34,35] to comply consistently with the requirement for stable operating equilibrium of recurrent artificial neural networks possessing billions of local feedbacks with time-varying delays, which may involve time-delays, as proved in [56,57]. It is thus believed this novel representation model may well yield new prospect for further innovative improvements and wider of applications. In addition, considerable empirical evidence in Biological Neuroscience has been established suggesting that sections of human living recurrent neuronal network, while performing specific reasoning tasks, get coupled [27,46,58,59] into certain optimizing mean field game-like cooperation or noncooperation. Thus, one possible modelling extension may be foreseen along the lines of mean-field game and optimal control theory [15,18,39].
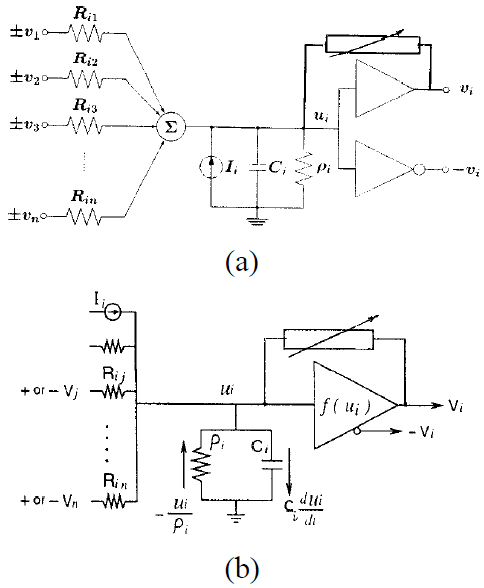
Figure 5: Schematic representation of the neuron unit in Hopfield networks based on physical electronics (a) as depicted in [54], Figure 1.3 in Sec. 1.2.5, where its essentially nonlinear dynamics is emphasized by its input-output characteristic of amplifiers and Khallil’s comprehensive Lyapunov stability analysis in Sec. 9.5.
An Enquiry: Neural Intelligence Versus Biology
Comparison Looks Back on Artificial and Living Neurons Versus Human Neuronal System
Science of neural networks, since the artificial neuron of McCullock and Pitts [1] via Hopfield’s neurons [3] to Hagen and co-authors [46] neural designs to Kasabov spiking-nerurons and ‘neucube’ evolving connectionist systems by Kasabov [25,26] to hybrid spiking models of neurons by Izhikevich [4,28] have undergone developments beyond any predictions put forward in due course. Even the most recent works by Hagen and co-authors, they begin rightly their book with this very first sentence: “As your read these words you are using a complex biological neural network. You have a highly interconnected set of some 1011 neurons to facilitate reading, breathing, motion and thinking. Each of your biological neurons, a rich assembly of tissue and chemistry, has the complexity, if not the speed of microprocessor. Some of your neural structure was with you at birth. Other parts have been established by experience.” ending the quotation. Apparently there is no any other indication for further step to approach and possibly meet the other side of complex neural networks spectrum, the computational one.
Living Neurons Versus Recurrent Artificial Neuron Nets
These authors have not found even an indication for any further step into the other side of complex neural networks the spectrum, human living neuronal networks, to which recurrent artificial neural networks should imitate. Yet, on the other side of this spectrum Science of Neurophysiology [5] has yielded insights, which are converging closer and closer to the approach via the novel science of Systems Biology. Though, it is true that those rather involved issues on the crossroad of the current findings with Kolmogorov’s representation superposition and Hilbert’s Thirteen Problem. Furthermore, as thoroughly discussed by David A Sprecher [53] in monograph [22], it appeared [27] these crossroad findings to yield emergence of certain rather delicate subtle issues, which are yet to be explored [6,12,22,58,59].
Concluding Remarks
An innovated model of the neuron unit has been derived on the grounds of the recent discoveries of essential indispensable glianeuron- cell co-existence symbiosis in human neurons. It has been obtained following Hopfield’s ideas envisaging the unit neuron as well as recurrent neural network on the grounds of analogue electronics model [23,30]. The new feature in the proposed model is the emulation of glia-neuron-cell symbiosis in terms of local feedback path, which may be static proportional one or timevarying and even varying as if controlled switching of nonlinear systems.
Though intelligent systems ought to emulate biological intelligence [48] as close as possible and so do artificial neural networks [12,34,42]. The underlying issues of the varying feedback are an open task yet to be explored since design synthesis of function NFBji( t, vj ) solved. This is a topic of future research endeavors along the lines of exploration as in works [35,50].
Acknowledgment
The author acknowledges the contributions by Prof. Kevin Warwick, Universities of Reading and of Warwick, UK, as well as their former doctoral students in the common endeavors to crossexplore the parallels between human neurons and recurrent artificial neurons.
Funding
This research has been supported in part by the Institute for Automation & Systems Engineering in School of Electrical Engineering & Information Technologies, Saints Cyril & Methodius University in Skopje, Karpos 2.
References
- McCuloch WS, Pitts W (1943) A logical calculus of the ideas immanent in nervous activity. Bull Math Biol 52(1-2): 99-115.
- Hopfield J (1982) Neural networks and physical systems with emergent collective computational properties. Proc Natl Acad Sci U S A 79(8): 2554-2558.
- Hopfield JJ (1984) Neurons with graded responses have collective computational properties like those of two-state neurons. Proc Natl Acad Sci U S A 81(10): 3088-3092.
- Izhikevich EM (2004) Which model to use for spiking neurons? IEEE Transactions on Neural Networks 15: 1063-1070.
- Guyton AC, Hall JE (2006) Medical Physiology (11th ,). Elsevier Sounders, Philadelphia, PA, USA, p. 54-58.
- Siljak DD (2008) Dynamic graphs. Nonlinear Analysis: Hybird Systems 2: 544-67.
- Sprecher DA (2017) From Algebra to Computational Algorithms: Kolmogorov and Hilbert’s Problem 13. Docent Press, Boston, MA, USA.
- Ashby WR (1947) Principles of self-organizing dynamic system. J Gen Psychol 37(2): 125-128.
- Kasabov NN (2014) Neucube: A spiking neuronal network architecture for mapping, learning and understanding spatio-temporal brain data. Neural Networks 52: 62-76.
- Zhao F, Zhang Y, Wang G (2018) A brain-inspired decision making model based on top-down biaising of perfrontal cortex to basal ganglia and its application to autonomous UAV exoplorationss. Congnitive Computation 10(2): 296-306.
- Sen Bhattacharya B, James S, Rhodes O, Sugiarto I, Rowley A, et al. (2018) Building a spiking neural netwrok model of the basal ganglia on SpiNNaker. IEEE Transactions on Cognitive & Developmental Systems 10(3): 823-836.
- Vershure PFMJ (2012) The distributed adptive control architetcture of the mind, brain, body nexus. Biologically Inspired Cognition Archive 1: 55-72.
- Hopfield JJ (1984) Neurons with graded responses have collective computational properties like those of two-state neurons. Proceedings of the National Academy of Sciences of the USA 81: 3088-3092,
- Hawking SW (2014) Conservation of information and estimation of time for black holes. ArXive, arXive-paper 1-3.
- Li T, Zhang JF (2008) Asymptotically optimal decentralized control for large population of multi-agent systems. IEEE Transaction on Automatic Control 53(7): 1643-1660.
- Ni YH, Zhang JF, Dimirovski GM (2018) An introductory review of time insonsistent stochastic optimal control. In Proceedings of the 37th Chinese Control Conference, Vuhan, 25-27 Chinese Association of Automation, Beijing, China, pp. 2528-2533.
- Wang BS, Zhang JF (2012) Mean field games for large population multi-agent systems with Markov jump parameters. SIAM Journal on Control and Optimization 50: 2308-2334.
- Dimirovski GM, Wang R, Yang B (2017) Delay and recurrent neural networks: Computational cybernetics of systems biology?. In Proceedings IEEE SMC 2017 on Systems, Man and Cybernetics, Banff, Canada. The IEEE, Piscataway, NJ, USA, pp. 906- 911.
- Fields R.D, Beth SG (2002) New Insights into neuron-glia communication. Science 556-562.
- Gurney K, Prescott TJ, Redgrave P (2001) A computational model of action slection in basal ganglia, Part I: A new functional anatomy. Biological Cybernetics 84(6): 401-410.
- Pfrieiger FW (2002) Role of glia in synapse development. Current Opinions in Neurobiology 12(5): 486-490.
- Fornito A, Zalesky A, Bulmore E (2016) Fundamentals of Brain Network Analysis. Academic Press, New York, USA.
- Kasabov N (2003) Evolving Connectionist Systems: Methods and Applications in Bioinformatics, Brain Study and Intelligent Machines. Springer Verlag, USA.
- Izhikevich EM, Edelman GM (2008) Large-scale model of mammalian thalamocortical systems. Proceedings of the National Academy of Sciences of the USA 105(9): 3593-3598.
- Izhikevich EM (2010) Hybird spiking modesl. Philosophical Transactions of Royal Society A 368(1930): 5061-5070.
- Haydon PG (2001) Glia: Listening and talking to the synapse. National Reviews of Neuroscience 2: 844-847.
- Hodgkin AL, Huxley AF (1952) A quntitative description of membrane current and its application to conduction and excitation in nerve. The Journal of Physiology 117(4): 500-544.
- Ikuta C, Uwate Y, Nishio Y (2011) Performance and features of multi-layer perceptron with impulse glial network. In: Proceedings of the 2011 International Joint Conference on Neural Networks. The IEEE, Piscataway, USA, pp. 2536-2541.
- Ikuta C, Uwate Y, Nishio Y (2012) Investigation of multi-layer perceptron with including glial pulse in two directions. In: In Proceedings of the 2012 IEEE International Symposium on Circuits and Systems. The IEEE, Piscataway, NJ, USA, pp.2099-2102.
- Protic SP (1990) Molecular and Functional Intercation of Neurons and Glia Cells. Gornji Milanovac, RS-SFRY “Decje Novine”.
- Dimirovski G, Warwick K (2018) From recurrent neural netwroks to human neuronal netwroks and back: A Computational Bio- Cybernetics. In Proceedings of the 14th International Conference ETAI-2018, Struga, Macedonia, 20-22 September 2018. Skopje, MK, Republic of Macedona.
- Dimirovski GM, Warwick K, Stefanovski JD (2019) Complexity Symbiosis of Glia-Neuron Cells and Computational Cybernetics of Hopfield Recurrent Network: Novel Neuron Model. In Proceedings IEEE SMC 2019 on Systems, Man and Cybernetics, Bari, Italy.
- Gurney K, Prescott TJ, Redgrave P (2001) A computational model of action slection in basal ganglia, Part II: Analysis and simulation of behavior. Biological Cybernetics 84(6): 411-423.
- Guyton AC, Hall JE (2006) Medical Physiology (11th edition). Elsevier Sounders, Philadelphia, USA, p. 54-58.
- Boldog E, Bakken TE, Hodge RD, Nowotny M, Aevermann BD, et al. (2018) Trascriptomic and morphophysiological evidence for a specialized cortical GABAergic cell type. Nat Neurosci 21(9): 1185-1195.
- Bensoussan A, Frehse J, Yam P (2013) Mean Field Games and Mean Field Type Control Theory, Springer Briefs in Mathematics. Springer Verlag, Heidelberg, Germany.
- Chiappalone M, Vato A, Berdondini L, Koudelka Hep M, Martinoia S (2007) Network dynamics and synchronous activity in cultured cortical neurons. Int J Neural Syst 17(2): 87-103.
- Moser EI, Roudi Y, Witter MP, Kentros C, Banhoeffer T, et al. (2014) Grid cells and cortical representation. Nat Rev Neurosci 15(7): 466-481.
- Nikolic D (2017) Why deep neural nets cannot ever match biological intelligence and what to do about it?. International Journal of Automation and Computing 14(5): 535-541.
- Sun Y, Zhao J, Dimirovski GM (2019) Adaptive control for a class of state-constrained high-order switched nonlinear systems with unstable subsystems. Nonlinear Analysis: Hybrid Systems 32: 91-105.
- Cagla E, Ben AB (2010) Regulation of synaptic connectivity by glia. Nature 468: 223-231.
- Buffo A, Rossi F (2013) Origine lineage and function cerebeller glia. Progess in Neurobiology 109: 42-63.
- Hagen MT, Demuth HB, Beale MH, Olando De Jesus (2012) Neural Network Design (2nd Edn). Oklahoma State University, Stillwater, OK, 2012.
- Edelman GM (2007) Learning in and from brain-based device. Science 318(5853): 1103-1105.
- Nikolic D (2015) Practopoesis: Or how life fosters a mind. Journal of Theoretical Biology 373: 40-61.
- Ikuta C, Uwate Y, Nishio Y, Yang G (2013) Multi-layer perceptron with propagation of glial pulse between learning and non-learning. In Proceedings of the 2013 IEEE International Symposium on Circuits and Systems. The IEEE, Piscataway, NJ, USA, pp.2107-2110.
- Warwick K (2016) Creating and Controlling Complex Biological Brains. In: Dimirovski GM (Edn.), Complex Systems: Relationships between Control, Communications and Computing. Springer International, USA, pp.133-148.
- Warwick K, Xydas D, Nasuto SJ, Becerra VM, Hammond MW, et al. (2010) Controlling a mobile robot with a biological brain. Defence Science Journal 60(1): 5-14.
- Warwick K, Nasuto S, Becerra V, Whalley B (2011) Experiments with an in-vitro robot brain computing with instinct. In Cai Y (Edn.), Lecture Notes in Computer Science, Springer Verlag, Berlin, USA, p. 1-15.
- Khallil HK (2002) Nonlinear Systems (3rd Edn). Apper Saddle River, NJ, USA.
- Jang JSR, Sun CT, Mizutani E (1997) Neuro-Fuzzy and Soft Computing; A Computational Approach to Learning and Machine Intelligence. Apper Saddle River, NJ, USA.
- Yang B, Wang R, Shi P, Dimirovski GM (2015) New delay-dependent stability criteria for recurrent neural networks with time-varying delays. Neurocomputing 151: 1414-1422.
- Yang B, Wang R, Dimirovski GM (2016) New delay-dependent stability neural networks with time-varying delays via a novel partitioning method. Neurocomputing 173: 1017-1027.
- Ashby WR (1947) Principles of self-organizing dynamic system. The Journal of General Psychology 37(2): 125-128.
- Maed C (1990) Neuromorphic electronic systems. Proceedings of the IEEE 78(10): 16-29.
- Huang TJ (2017) Imitating the brain with neurocomputer: A “new” way towards artificial general intelligence. International Journal of Automation and Computing 14(5): 5520-531.
- Keener J, Sneyd J (1998) Mathematical Physiology. Springer-Verlag, New York, USA.
- Marks P (2008) Rat-brained robots take their first steps. New Scientist 199(2669): 22-23.
- Yeargers EK, Shonkwiler RW, Herod JV (1996) An Introduction to Mathematical Biology with Computer Algebra Models. Boston, MA, USA.