Research Article
Creative Commons, CC-BY
Establishing Practical Quality Indicators for Improving Care of Newborns in Low-Middle Income Countries
*Corresponding author: Bernhard Fassl, Center for Global Health Innovation, College of Graduate Studies Profesor of Pediatrics, College of Medicine, USA.
Received: September 01, 2023; Published: September 11, 2023
DOI: 10.34297/AJBSR.2023.20.002673
Abstract
Background: In developing countries, nearly 50% of all childhood deaths occur in the neonatal period, most commonly in the first 2 days of life. A large proportion of these deaths occurs in health facilities and is due to inappropriately recognized and managed birth asphyxia, infections and low birth weight. A lack of quality indicators makes it challenging to quantify and track care quality, and to measure the impact of Quality Improvement (QI) interventions in low resource settings.
Objectives: The goals of this study were to (1) develop a set of newborn care Quality of Care (QoC) measures that are feasible and applicable in resource limited settings and (2) utilize these QoC measures to drive QI interventions in a community level hospital in rural India.
Methods: Potential QoC measures representing key newborn care processes were extracted through a literature review using pre-specified criteria and from WHO guidelines, then categorized based on level of scientific evidence. Through a modified Delphi technique, using a consensus panel of local and international experts and 2 rounds of review, key QoC measures, deemed appropriate for newborn care were selected and rated for feasibility. We then used selected measures to assess the community hospital compliance at baseline (2014) and during implementation of a comprehensive newborn QI program (2015 to 2018). QI interventions included tracking key QoC measures, feeding back monthly performance data to frontline providers, and using PDSA cycles to identify and address persistent challenges. Run charts were used to assess change over time and T-test to compare performance between pre/post intervention.
Results: Of 27 potential QoC measures identified from literature review and WHO guidelines, 10 were selected by the expert panel and grouped into 3 categories: (1) QoC measures for newborn care immediately after delivery and (2) QoC measures for general and (3) low-birth-weight newborn care. We recorded compliance with QoC measures in 226 care encounters pre-intervention and in 717 encounters during a 4-year QI intervention. Compliance across all QOC measures was low at baseline (All score average 38%; Range: 0-100%) and improved in most areas following a QI intervention (62%; Range: 0-100). Measurements showed variability between intervals attributable to changes in staffing and care organization.
Conclusions: This study identified feasible evidence based newborn care quality measures for low resource settings which can be used for trending quality of care. A quality improvement intervention resulted in significant and sustained improvement of care quality in most areas.
Introduction
Annually 5.4 million live-born children die before reaching their fifth birthday. Forty six percent or 2.5 million of these deaths occur in limited resource setting and in the first 28 days of life known as the neonatal period. In addition, 3.2 million children were reported stillborn. It is estimated that approximately 1 million of these stillbirths are due to intra-partum complications. While there has been progress in reducing the number of overall child deaths worldwide, this progress has been much slower among newborns [1-4]. Neonatal deaths now account for 46% of all under 5y child mortality [5].
According to the WHO, the most common direct causes of death in the neonatal period are prematurity and Low-Birth Weight (LBW) complications (16%), birth asphyxia 11% and neonatal sepsis (11%) [6]. More than 80% of neonatal deaths occur in LBW babies - with a recent shift in the timing of these deaths closer to the time of birth [7-9]. Evidence-based, cost-effective interventions that directly address the neonatal causes of death are well known, and if implemented on a large scale with adequate population coverage, a large number of these deaths could be prevented [10, 11]. However, barriers to deliver evidence-based care for newborns in limited settings persist, leading to poor outcomes. Poor outcomes are commonly the result of multiple factors, including limited access to healthcare facilities, limited skills of healthcare staff, limited equipment availability, lack logistical support, and suboptimal local leadership [11,12].
Despite recent increases in both financial and personal investments in health facilities, the quality of health service delivery has remained a critical problem in many countries, including India [13]. Ensuring coverage of interventions that directly target the leading causes of neonatal death are among the highest priorities among global policy makers. Effective application of guideline based best practice has remained problematic in many settings worldwide [14-16]. A major challenge in health program implementation that specifically target causes of neonatal deaths through care quality interventions is the lack of universally applicable, valid and feasible quality indicators for optimal neonatal care [17]. Previous publications have recognized the importance of Quality Care (QoC) measures on patient outcomes, but actual measurement of health care quality is a major challenge, particularly in Low-Middle Income Countries (LMICs) [18-21]. A variety of newborn QoC measures, as well as general guidelines, are available in the literature but practical use of these measures to benchmark and improve health care delivery across health systems has not yet been accomplished. In addition, many of the existing QoC measures are centered around patient outcomes rather than on evidence-based care processes [22-27]. Reduction in newborn mortality will require translation of existing guidelines into feasible QoC measures that could be broadly used in LMICs to monitor and benchmark care delivery, identify improvement opportunities and guide future health interventions [28].
The purpose of this study is to develop a set of key QoC mea sures for newborns that are feasible and applicable in resource limited settings to drive care process improvements. Specifically, we sought to (1) identify feasible evidence based QoC measures that are aligned with both national and international health priorities, and (2) utilize these measures to assess health care performance of a rural hospital in India, pre- and post-implementation of a new Quality Improvement (QI) intervention.
Methods
Study Location
This study took place in Shree Chhotubhai A. Patel Hospital (CAPH) in Vadodara district in the state of Gujarat, India. The hospital serves as the local governmental Community Health Center (CHC) and is the designated governmental health facility for Sinor Taluka (administrative unit). CAPH provides basic medical services to a population of approximately 80,000 and consists of a 50-bed general adult and labor unit as well as a 35-bed pediatric unit. Hospital core staffing includes two senior physicians (10+ years’ experience), 2-3 junior physicians (<2 years’ experience), 11 nurses and 14 helpers known as “wardboys”. With regard to specialty services, 1 obstetrician is consistently available while pediatric and surgical care are available only intermittently. The hospital is located in a rural farming community and serves as the primary referral center in the area. CAPH is managed by the Shakti Krupa Charitable Trust (SKCT), a local no governmental organization, which operates the hospital as a public-private partnership operation together with the health ministry of Gujarat. The University of Utah (UU) has a training and clinical partnership with CAPH and provides technical/ clinical assistance, education and professional and programmatic development to local providers. This QI study was approved by the Institutional Review Board (IRB) of the University of Utah and was carried out with the permission of CAPH and in collaboration with the Gujarat Pediatric Society.
Study Design
This study was a quality improvement study in which we utilized the existing hospital resources, medical and administrative infrastructure to operationalize neonatal care improvement interventions following iterative PDSA improvement cycles. The study had 2 distinct phases. Phase 1: Definition of QoC measures; Phase 2: Application of QOC measures in a clinical setting during a newborn quality of care improvement.
Phase 1 - Definition of QOC Measures - Jan -July 2014: Identification of quality measures: We assembled a multidisciplinary team, consisting of 5 frontline clinical care providers with daily exposure to routine neonatal care issues (one pediatrician, two general physicians, one nurse, and one ward boy), one hospital administrator, one nationally recognized pediatric expert and health policy representative (President of the Gujarat Pediatric Society - pediatrician in practice) and 1 international pediatric advisor (BF) who primarily facilitated the consensus process. Our team performed a literature review for neonatal care guidelines to identify key neonatal care processes and define potential quality measures that are: aligned with national and international health priorities, evidence based, applicable in low resource settings, and applicable in rural India. The guidelines reviewed included the national Indian neonatal care guidelines, WHO and UNICEF core publications, as well as Gujarat state level health policy guidelines [29-38].
This review yielded 27 measures (Table 1) which were selected for an in-depth discussion by the team. We performed 2 rounds of review, during which all measures were ranked with regards to feasibility and applicability using a 3-point Likert scale. (Not-moderately- highly. Feasible/applicable). After each review, the 5 lowest performing measures were selected for discussion to be excluded. Elimination of measures was based on a consensus agreement within the group. Furthermore, the group agreed to preferentially select measures that primarily target the 3 major causes of neonatal death: prematurity, low birth weight, and intrapartum complications. During the review process and ensuing discussions, some measures were refined from their original definition or were combined to serve as cluster measures. Ultimately, the team achieved consensus on 10 newborn QoC measures, which were grouped into 3 categories (Table 2) based on whether they were appropriate for immediate newborn care, routine newborn care or for and for low-birth-weight (LBW) newborns.
Phase 2: Implementation of Quality Improvement Project and Application of QoC Measures During a Quality Improvement Intervention to Improve Neonatal Care Delivery and Support Best Practice (June 2014 - 2019): We assembled a multidisciplinary QI team which consisted of representatives from each of the professional groups providing care: hospital administration, physicians, nurses, ward boys. The local QI was mentored by the University of Utah project team which followed the QI framework methodology designed by the Institute of Medicine (IOM) outlining 6 aims and 10 rules for care delivery redesign [39] and utilized Six Sigma’s DMAIC (define-measure-analyze-improve-control) implementation model for this project in India [40]. We designed improvement interventions to support best practice and address gaps and build capacity in 6 domains of health care delivery. Summary of key interventions is outlined in (Table 3).
After obtaining baseline (Jan May 2014) performance of CAPH with the 10 QoC measures, the QI team initiated a hospital based newborn care QI project in May/June 2014 and assessed compliance with QoC measures in 6-12 month intervals and assisted the local medical and administrative leadership with design of PDSA cycles and ongoing improvement interventions. In addition to regular QoC assessments, educational, equipment upgrade and health systems interventions, we performed focus groups with the physicians, nurses, wardboys and administrations to understand perceived barriers to improvement in newborn care. Focus groups were held in conjunction with staff meetings during which the current rate of compliance with a particular care measure was presented graphically. Current measures were discussed as a group and goals were set for the next 6 months. PDSA improvement cycles were applied every 6 months and are ongoing (Tables 4,5).
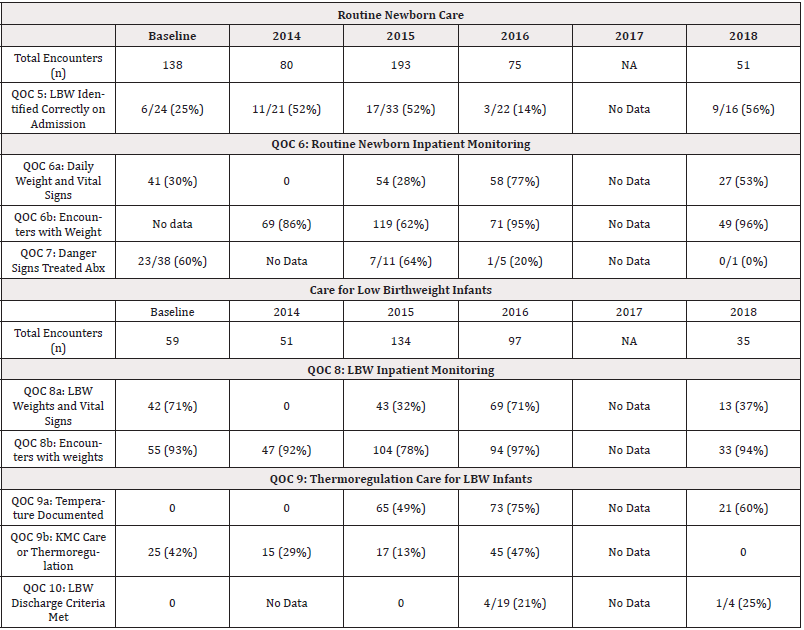
Table 5: Trends in Compliance among the health care team for Newborn and Low Birthweight Care (QOC 5-10) following dissemination of the Newborn Care Queslity Measures.
Throughout the intervention, we only used local resources and did not provide funding or introduce external resources from overseas to facilitate improvement, such as extra staff, external funds, or additional equipment. Our team operated within the pre-existing hospital budget for staff and equipment. All changes in staff and equipment reallocation were due to internal re-prioritization of funds and human resources.
Data Collection
Utilizing the hospital quality team previously described in conjunction with visiting faculty, residents and students from the University of Utah, we collected QoC data at baseline and during the QI implementation period, performed workflow and gap analyses and PDSA improvement cycles to address care gaps. Compliance with QoC was used to benchmark CAPH and to serve as tools for monitoring the impact of the QI interventions.
To determine CAPH performance, we performed direct observation of care delivery at the patient’s bedside using a standardized data collection tool checklist representing compliance with QoC identified in the review process. Our team developed a checklist for the delivery room and for pediatric care on the ward and newborn care unit. Data was collected by visiting medical students and pediatric residents from the University of Utah who received training by the US-based project leaders prior to data collection. Time intervals of data collection were selected by convenience and were based on the availability of medical students or residents for global rotations, approximately every 6 months in the initial project stage and every year during the maintenance phase. Data collection was not performed by members of the QI team in order to minimize bias.
Agregate baseline (prior to QI interventions) performance was determined for the pre-intervention period (Ja Jun 2014), while performance post-intervention time intervals (Jun 2014 - present) was assessed over time, using run charts depicting compliance with each individual QoC measures. We also analyze the compliance data in a pre/post intervention comparison using a T-test to determine significance.
Results
During the study period, a total of 1294 births took place at CAPH according to the hospital registrar. Throughout the study period from 2014 to 2018, the characteristics of the babies delivered remained consistent. In 2014, there were 382 deliveries, the average birthweight was 2540 grams. Forty percent (154) of babies delivered were LBW with birth weight less than 2500 grams, and 7% (27) of babies weighed less than 1800 grams. There were 4 still births and 1 neonatal death recorded. In 2015 there were 538 deliveries recorded, an average birthweight of 2593 grams. There were 223 LBW babies delivered, accounting for 41.4% of all deliveries. Twenty-one babies weighed less than or equal to 1800 grams (4% of all deliveries. There were 5 stillbirths and 3 neonatal deaths recorded. In 2016, there were 374 recorded deliveries, with an average birthweight of 2604 grams. There were 152 LBW babies delivered, accounting for 40.6% of all deliveries. Fourteen babies had a birthweight of less than or equal to 1800 grams (3.7% of all deliveries). There were 5 stillbirths and 2 neonatal deaths recorded.
Compliance Pre and Post QI Intervention Results
From May of 2014 to August 2018, medical students trained in data collection directly observed care delivery in the newborn unit and completed a standardized checklist of the essential newborn care provided to infants. Data was collected using a standardized data collection tool. We report provider compliance and trends in the quality of care over time. In the evaluation phase, which took place at distinct data point collection dates from May 2014-June 2016, a total of 718 care encounters during 5 distinct data collection periods were performed. At baseline compliance with the QoC was low (0%). Following a combined training and quality improvement intervention for hospital staff, care quality varied widely among the 10 different benchmark measures. Overall, there was an improvement in the care provided to low birthweight infants and delivery room management, however, there remains clear deficits in the quality of care provided to the neonatal population. Graphs 1-2 depict the QoC trends and ongoing challenges associated with maintaining focus on quality care improvement (Graphs 1,2).

Graph 2:
Graphs 1 and 2: QOC 5 and QOC 8: Appropriate management of Low Birthweight (LBW) newborns. Compliance with identification and management of high-risk low birthweight infants has steadily improved over time. One multifactorial challenge remains keeping low birthweight babies admitted to the hospital until weight>1800g, established feeding and weight gain x 3 days, normal temperature.
Discussion
The purpose of this project was to create practical, standardized and generalizable benchmarks of neonatal care in low-middle income areas. These benchmarks were created to measure evidence- based care processes during and after implementation of health systems strengthening programs with the goal of improving neonatal care. Benchmarks were applied in conjunction with a quality improvement intervention to track progress in real time and provide health process feedback loops. To our knowledge this is the first study designed to define universally applicable newborn QoC benchmarks for use during a DMAIC based quality improvement intervention.
Process Data Driven Approach to Close Quality Gaps in Resource Poor Settings
Our team was successful in (1) demonstrating that a consensus process can be applied to determine evidence based QoC benchmarks and that (2) they can be integrated within a hospital system to measure quality over time, track progress - or lack thereof and assist with ongoing performance evaluation of a care process. Our study utilized QoC benchmarks to track progress and measure effectiveness of a variety of interventions targeted at improving care quality for newborns. These measures proved to be a valuable tool to guide hospital administrators toward data driven quality care interventions.
Basic, evidence based neonatal care processes are well defined in global and Indian health guidelines. However, numerous studies describe that high quality services for newborns have the greatest gaps in communities that need them most [41,42]. Recent publications detail shortfalls in care services during delivery and newborn care. Critical health system bottlenecks were identified with regards to leadership and governance, financing, health workforce, essential commodities and equipment and care service quality monitoring among others. As a result, health policy planning is evolving to prioritize health systems level interventions [43,44].
While available evidence and expert consensus of what constitutes appropriate neonatal care in health facilities in LMIC is abundant [45,46], operationalization of general guidelines in daily practice has remained challenging. Scaled up interventions of newborn care implemented country wide have often fallen short of expected successes [47] and birth asphyxia, low birth weight and infections continue to account for 85% of all neonatal deaths worldwide [48]. In LMICs, only half of mothers and newborns receive postnatal care - in a country analysis of the 10 countries with the highest neonatal mortality, about 50% of newborns had access to “skilled” care but only 1-6% of patients received the full package of monitoring and care recommended interventions for improved outcomes [49,50]. We believe that universal application of nQOC measures could provide critical care process information to assist stakeholders in improving service delivery for newborns at the health facility and policy level.
Management of the Newborn Immediately After Delivery
During our study period, it proved “easiest” to achieve gains in the care delivery to the newborn immediately after birth. Our combined interventions resulted in increased competency (data not shown in this publication) based on standardized evaluation methods developed in conjunction with training programs (i.e., Helping babies Breathe). Provider performance in the delivery room improved significantly after application of the QI framework described in this paper. These gains could largely be maintained over time and direct observation of care in 2018 showed that many of the care interventions now have become part of the “institutional memory” and have been adopted by frontline health workers as the new standard of care. The number of observations of advanced measures (bag and mask ventilation) were small and our study is unable to determine the significance of intermittent decreases in application of advanced measures. We hypothesize that due to staff turnover between QI cycles and the presence of staff members who lack understanding and critical skills, bag and mask ventilation was not universally available and applied.
Routine Neonatal Care and Low Birth Weight Qoc
While care interventions immediately after birth are well protocolized, time limited and confined to the delivery room, care delivery to newborns in a general ward is a much more complex task. Evidence behind the selected QoC benchmarks is strong, operationalization and improvement in performance proved much more difficult in a clinical setting: Even basic interventions, such as obtaining vital signs and daily weights, were a challenging task. We believe that this is due to the following factors: First, obtaining vital signs by hand using a stethoscope or visual inspection of the child to observe respirations is a time-consuming effort and requires a cooperative child. Health facilities in developing countries are commonly understaffed, and the nurse-to-patient ratio is very low. At CAPH, depending on patient load, the ratio is often 1 nurse for every 30-40 inpatients. In addition to patient assignments, the nurses are needed to attend to duties in the emergency room and delivery room. During workflow analysis, the QI team concluded that obtaining vital signs multiple times per day is simply not feasible in the current care setting and with the current staffing level. We believe that there is room and opportunity to introduce innovative low-cost technological devices which can assist the staff to obtain vital signs. Devices which measure temperature, heart rate, respiratory rate, oxygen saturation are available commercially but to date the cost is prohibitive to universal application in LMICs. Second, we observed that doctors who make clinical decisions did not ask for vital signs and were not trained and used to using vital signs in their medical decision making, thus providing little incentive for the nurse to obtain and document them.
The effect of educational interventions was not only low with regards to vital signs but was also limited for interventions which address a more complex patient condition and medical decision making, or which required a variety of equipment, staff interaction and medical infrastructure. For example, provision of bag mask ventilation is based on a simple “yes - no” algorithm during assessment of the breathing status of a newborn. In contrast, the decision of how to maximize weight gain in LBW infants is more complex: Choosing appropriate feeding goals, weighing options such as expressing breastmilk vs formula, calculating intake as mL per kg bodyweight per day, and applying strategies to improve feeding efficiency proved to be a highly complex and time-consuming task for the medical team to execute. Using repeated PDSA cycles and gap analyses our team identified many of the actual root causes of poor QoC performance, however mitigation requires a system level approach as described by Enweronu-Laryea, et al. [44].
Our QI team also experienced the effect cultural barriers have on QoC benchmarks. Kangaroo Mother Care (KMC) especially for low-birth-weight infants is an Evidence level A recommended intervention and has been shown to be associated with improved outcomes and rediced mortality. Patient adherence to KMC despite repeated instruction was low - while in other regions in the world, skin to skin contact is considered normal part of newborn care, it is an unusual component of patient-infant interactions in this particular social/cultural background.
Challenges for Application of nQOC Measures
A major challenge during this study included staff perception - in that care process data would be utilized to “punish” front line health workers, a concept commonly applied in India: The concept that data driven care planning, using standard QI tools such as gap analysis tools, work process mapping and pareto charts are utilized for quality improvement rather than punishment was novel for the local team and resistance to this approach was palpable especially at the beginning of the project.
Our feedback loops also exposed system challenges which could not be addressed at the local level with targeted medical, educational or equipment intervention but would require a significant restructuring of the health service delivery system. Our effort revealed continued barriers which exist in rural health facilities in India and probably in other LMICs - highlighted by the lack of improvement in certain QoC measures - most notably due to a lack of adequate numbers of staff to execute some of the care tasks (multiple daily assessment of vital signs), excessive workload and lack of a quality-of-care oriented hospital governance system. An additional challenge was to manage disagreements between health staff and nQOC measures. Initially, several senior level physician staff were opposed to accepting and following nQOC guidelines as this significantly deviated from their established standard practice. In contrast, mid- and lower-level health staff (nurses and ward boys) were generally highly supportive and ready to adapt new care practices and readily embraced the new skills and showed readiness to apply them in daily practice. This initially resulted in disagreements over patient care, including the delivery room especially with applying bag and mask ventilation quickly (within 1 minute) to apneic infants. These conflicts were often resolved on the basis of hierarchy rather than appropriate evidence-based care. This challenge decreased over time as senior staff “got more used” to new care pathways and data feedback during staff meetings about improved newborn outcomes provided reassurance.
Hospital Capacity and Readiness for Data Driven QI
Even though the government mandates reporting of many clinical and administrative data to central authorities, clinically relevant aggregate data and analysis of this information is not shared back with the respective institution. Due to the lack of feedback loops, facilities are unable to view and act on their own performance data and are trapped in a cycle of service delivery without clearly understanding the trends and current status to identify areas of improvement or progress.
Our team observed that hospital administrators initially lacked the capacity of obtaining and preparing relevant care process data for self-directed PDSA drive QI cycles. Training the hospital administration to embrace the conceptual framework of quality improvement and to change the operating culture from a punitive model for poor outcomes to a system-change approach required a significant and multi-year effort of role modeling. The governing style, reward and punishment system, is a unique characteristic of each facility, region, nation and needs to be taken into consideration for any QI project which takes place at an institutional level and was an enormous challenge in the beginning phase.
After overcoming these initial challenges and realizing the value in measuring and trending care process measures, the local leadership readily embraced the concept of data-driven decision making at the hospital level and set in motion efforts to facilitate and sustain these efforts: Through a public-private-partnership program the hospital developed a EMR system which generates monthly reports for use by the local leadership and is now reviewed with the staff on an annual basis in a facilitated hospital performance review. In addition, care process analysis paired with work flow evaluation prompted the hospital leadership to reassign roles and responsibilities among staff to better complete the clinical tasks and manage patients. This included expanding the roles of “wardboys” - trained helpers without formal medical education and promoting nurses to take on a more active leadership role.
Limitations
This project has several limitations:
a) This study was conducted at a single center in rural India and findings especially with regards to methods on achieving improvements in care quality may not be generalizable to other hospitals in India or regions in the world. We believe that each institution has its own “institutional memory” and intrinsic challenges with regards to achieving maximum care quality. The results of this study are not intended to define the only way to achieve improvement in care quality, but are rather meant to describe the general approach an institution or health system can take in order to standardize care, define shared goals and apply PDSA based strategies to achieve improvement over time.
b) We are unable to quantify the effect of the observer on the outcome: Direct observation of care and completion of a checklist may alter staff behavior toward improved compliance. To minimize this effect, observations were completed by mostly 1st year medical students who received an introductory training course prior to leaving for India but were otherwise not trained or skilled to directly interfere with the care process. We believe however that the observation effect was only minimal, because of the high frequency of visiting US medical students present in this environment and the relative ease with which they were absorbed into the daily operations.
c) The study location was a government hospital which is operated as a public private partnership. We cannot assume that all facilities in the government sector are equally responsive to PDSA cycles.
d) In this study we excluded important evidence-based quality of care measures based on local expert consensus as they were considered less feasible and applicable in rural India but may be of greater value in other locations and settings. We recommend that other institutions consider a wider range of quality measures applicable to the local circumstances. The initial list of QoC prior to review - see (Table 3).
Conclusion
This study identified evidence based neonatal QoC benchmarks which can be operationalized at a health institution level. Even though the study was conducted in a single center in India, we believe that the QoC benchmarks apply to any newborn in the world and application of these benchmarks is feasible and desirable in any LMIC setting. This study shows that utilization of QoC benchmarks to direct QI interventions is a feasible and effective method to guide hospitals toward data driven, outcome-oriented interventions. Difficulties in achieving certain QoC benchmarks and maintaining program compliance were evident at CAPH during this 4-year time period. Challenges related to frequent staff turnover, lack of consistent oversight by trained personnel, and time from initial training are contributing factors to inconsistent program compliance. Further evaluation of the potential barriers and logistical challenges to program compliance is needed.
Acknowledgement
We would like to acknowledge the Shakti Krupa Charitable Trust, especially Mr. Jeetentra Patel (chairman), Mrs. Meghna Patel, Mr. Ashok Patel, Mr. Pranav Brahmbhatt and all CAPH staff for their dedication and contributions to this project. We thank the Dinesh and Kalpana Patel Foundation, Kiran and Pallavi Patel Family Foundation and Sorenson Legancy foundation for their program support. We thank the University of Utah, Department of Pediatrics and Center for Medical innovation for their contributions to this program.
References
- (2013) The UN Inter-Agency Group for Child Mortality Estimation. Levels and trends in child mortality: report 2013. New York: UNICEF.
- Lawn JE, Yakoob MY, Rachel A Haws, Tanya Soomro, Gary L Darmstadt, et al. (2009) Haws RA: 3.2 million stillbirths. Epidemiology and overview of the evidence review. BMC Pregnancy Childbirth 9(Suppl 1): S2.
- Bhutta Z A, Darmstadt G L, Lawn J E (2009) Stillbirths – the global picture and evidence-based solutions. Executive summary for the BMC Pregnancy and childbirth supplement.
- Stanton C, Lawn JE, Stein (2005) C: No cry at birth: global estimates of intrapartum stillbirths and intrapartum related neonatal deaths. Bull WHO 83(6): 409-417.
- Oestergaard M Z, Inoue M, Yoshida S, Wahyu Retno Mahanani, Fiona M Gore, et al. (2011) United Nations Inter-Agency Group for Child Mortality Estimation and the Child Health Epidemiology Reference. Neonatal mortality levels for 193 countries in 2009 with trends since 1990: a systematic analysis of progress, projections, and priorities. PLoS Med 8(8): e1001080.
- Liu L, Oza S, Hogan D, Jamie Perin, Igor Rudan, et al. (2015) Global, regional, and national causes of child mortality in 2000-13, with projections to inform post-2015 priorities: an updated systematic analysis. Lancet 385(9966): 430-440.
- Lawn J E, Blencowe H, Oza S, Danzhen You, Anne C C Lee, et al. (2014) Every newborn: progess, priorities and potential beyond survival. Lancet 384(9938): 189-205.
- Lawn J E, Davidge R, Paul V K, Severin von Xylander, Joseph de Graft Johnson, et al. (2013) Born too soon: care for the preterm baby. Reprod Health 10(suppl 1): S5.
- Victoria C G, Aquino E M, do Carmo L M, Carlos Augusto Monteiro, Fernando C Barros, et al. (2011) Maternal and child health in Brazil. Progress and challenges. Lancet 377(9780): 1864-1876.
- Bhutta Z A, Das J K, Bahl R, Joy E Lawn, Rehana A Salam, et al. (2014) Can available interventions end preventable deaths in mothers, newborn babies, and stillbirths and at what cost? Lancet 384(9940): 347-370
- Darmstadt G L, Bhutta Z A, Cousens S, Taghreed Adam, Neff Walker, et al. (2005) Evidence-based, cost-effective interventions: how many newborn babies can we save? The Lancet 365(9463): 977–988.
- (2014) [Partnership for Maternal, Newborn & Child Health Technical Paper]. Geneva: World Health Organization.
- Sharma G, Mathai M, Dickson K E, Andrew Weeks, G Hofmeyr, et al. (2015) Quality care during labour and birth: a multi country analysis of health system bottlenecks and potential solutions. BMC Pregnancy and Childbirth 15(Suppl2): S2.
- Lozano R, Wang H, Foreman K J, Julie Knoll Rajaratnam, Mohsen Naghavi, et al. (2011) Progress towards Millennium Development Goals 4 and 5 on maternal and child mortality: an updated systematic analysis. Lancet 378(9797): 1139–1165.
- (2013) UNICEF/WHO. Every Newborn: An action plan to end preventable newborn deaths.
- (2013) UNICEF. State of the world's children. UNICEF, New York, NY.
- Bhutta Z A, Salam R A, Lassi Z S, Anne Austin, Ana Langer, et al. (2014) Approaches to improve quality of care for women and newborns: conclusions, evidence gaps and research priorities. Reprod Health 11(Suppl 2): S5.
- (2006) WHO: Quality of care: a process for making strategic choices in health systems.
- Raven J, Hofman J, Adegoke A, van den Broek N (2011) Methodology and tools for quality improvement in maternal and newborn health care. Int J Gynaecol Obstet 114(1): 4-9.
- Moxon G S, Ruysen H, Kerber J K, Agbessi Amouzou, Suzanne Fournier, et al. (2015) Count every newborn; a measurement improvement roadmap for coverage data. BMC Pregnancy Childbirth 15(S2):S8.
- van den Broek N R, Graham W J (2009) Quality of care for maternal and newborn health: the neglected agenda. BJOG 116(Suppl 1): 18-21.
- Raven J, Hofman J, Adegoike A, Nynke van den Broek (2011) Methodology and tools for quality improvement in maternal and newborn health care. Int. J Gynecol Obstet 114: 4-9.
- Donabedian A (1988) The quality of care. How can it be assessed? JAMA 260(12):1743-1748.
- Raven J H, Tolhurst R J, Tang S, Nynke van den Broek (2012) What is quality in maternal and neonatal health care? Midwifery 28(5): e676-683.
- (2006) World Health Organization: Quality of care: A process for making strategic choices in health systems. Geneva: WHO.
- Tuncalp O, Were W M, MacLennan C, O T Oladapo, A M Gülmezoglu, et al. (2015) Quality of care for pregnant women and newborns s- the WHO vision. BCOG 122(8): 1045-1049.
- (2013) WHO. Consultation on Improving measurement of the quality of maternal, newborn and childcare in health facilities. Ferney Voltaire, France. ISBN 978 924 150741 7.
- (2010) International Institute for Population Sciences (IIPS), District Level Household and Facility Survey (DLHS-3), 2007-08: India, IIPS, Mumbai, India.
- World Health Organization. Recommendations on Newborn Care. WHO reference number: WHO/MCA/17.07.
- (2000) WHO. Integrated management of Pregnancy and Childbirth. ISBN 92-4-154587-9.
- (2010) WHO. Essential Newborn care course.
- National Health Mission India. Ministry of Health and Family Welfare.
- UNICEF: Integrated management of neonatal and childhood illnesses (IMNCI).
- (2013) WHO recommendations on postnatal care of the mother and newborn. ISBN: 978 92 4 150664 9.
- (2015) WHO, UN Populations Fund, UNICEF, World Bank. Pregnancy, childbirth, postpartum and newborn care. A guide for essential practice (3rd edition). ISBN 978 92 4 154935 6.
- (2009) WHO: Recommended interventions for improving maternal and newborn health. WHO/MPS/07.05.
- (2013) WHO Pocketbook of hospital care for children. 2nd edition.
- (2016) India Rural Health Mission: Guideline for standardization of labour rooms at delivery points. April.
- (2001) Institute of Medicine. Crossing the Quality Chasm: A New Health System for the 21st Century. Washington, DC: The National Academies Press.
- American society for Quality.
- Lawn J E, Kinney M V, Black R E, Pitt C, Cousens S, et al. (2012) Newborn survival: a multi-country analysis of a decade of change. Health Policy and Planning 27(Suppl 3): iiii6-iiii28.
- Spector J M, Agrawal P, Kodkany B, Lipsitz S, Lashoher A, et al. (2012) Improving quality of care for maternal and newborn health: prospective pilot study of the WHO safe childbirth checklist program. PLoS One 7(5): e35151.
- Sharma G, Matthews M, Dickson K, Andrew Weeks, G Hofmeyr, et al. (2015) Quality care during labour and birth: a multi country analysis of health system bottlenecks and potential solutions. BMC Pregnancy and Childbirth 15(Suppl 2): S2.
- Enweronu Laryea C, Dickson K, Moxon S, Aline Simen Kapeu, Christabel Nyange, et al. (2015) Basic newborn care and neonatal resuscitation: a multi country analysis of health system bottlenecks and potential solutions. BMC Pregnancy Childbirth 15(Suppl 2): S4.
- Dickson E Kim, Kinney V Mary, Moxon G Sarah, Ashton Joanne, Zaka Nabila, et al. (2015) Scaling up quality care for mothers and newborns around the time of birth: an overview of methods and analyses of intervention specific bottlenecks and solutions. BMC Pregnancy Childbirth 15(Suppl 2): S1.
- (2011) The Partnership for Maternal Health, Newborn and Child Health: Essential Interventions, Commodities and Guidelines for Reproductive, Maternal, Newborn and Child Health.
- Paudel D, Shrestha IB, Siebeck M, Eva Rehfuess, et al. (2017) Impactof the community-based newborn care package in Nepal: a quasi-experimental evaluation. BMJ Open 7: e015285.
- (2014) WHO, UNICEF: Every Newborn: An action plan to end preventable newborn deaths. Geneva: World Health Organization.
- (2014) WHO-CHERG provisional estimates.
- Requejo J, Cesar V, Bryce J (2015) Countdown to 2015 Report. Maternal, newborn and child survival. UNICEF and World Health organization. ISBN: 978-92-806-4814.