Research Article
Creative Commons, CC-BY
Did The Implementation of Government Mandates Reduce COVID-19-Related Mortality Rates?
*Corresponding author:Tilahun Emiru, Assistant Professor of Economics, Lake Forest College, USA.
Received: November 25, 2023; Published: January 30, 2024
DOI: 10.34297/AJBSR.2024.21.002835
Abstract
The global response to the COVID-19 pandemic witnessed an array of public health interventions aimed at curtailing the virusβs spread and saving lives. Notable among these were mask mandates and vaccine requirements. This study utilizes quarterly statelevel panel data from the United States spanning 2020-2021 to estimate the effect of vaccine and mask mandates on COVID-19- related mortality rates.
Introduction
The global response to the COVID-19 pandemic witnessed an array of public health interventions aimed at curtailing the virus’s spread and saving lives. Notable among these were mask mandates and vaccine requirements, which sparked debates regarding their efficacy and potential political motivations. As the discourse on these interventions intensified, researchers explored the nuanced effects, questioning whether decisions were driven by evidence driven research findings or political considerations. This study seeks to contribute to the ongoing dialogue by examining the impact of mask and vaccine mandates on COVID-19 mortality rates in the United States.
Previous research has explored the effectiveness of public health interventions in combating COVID-19. Meta-analyses by Chu, et al. (2020) and Liang, et al. (2020) provided insights into the positive effects of physical distancing, face masks, and eye protection [1,2]. Asadi, et al. (2020) investigated mask efficacy in reducing particle emission, while Howard, et al. (2021) also supported the reduction of respiratory particle transmission through mask-wearing [3,4]. Guerra & Guerra (2021) and Motallebi, et al. (2022) examined the impact of mask mandates at different levels, yielding varying results. Ahmad, et al. (2021) and other studies highlighted the overall consensus on the effectiveness of using masks. In terms of immunities and death rates, Gazit, et al. (2021) compared natural and vaccine-induced immunity, emphasizing the robustness of natural immunity [5-8]. Acton, et al. (2022) demonstrated the effectiveness of vaccine mandates at the college level in reducing COVID-19 cases and deaths [9]. The existing literature underscores the multifaceted nature of interventions and their impact on virus transmission and mortality.
This study utilizes quarterly data from all fifty states of the United States and the District of Columbia over 2020-2021. Incorporating vaccine and mask mandates, along with demographic, social, and clinical covariates in a multivariate regression analysis, the research assesses the association between vaccine and mask mandates and COVID-19-related death rates.
We find a negative and statistically significant relationship between vaccine mandates and mortality rates. Periods when vaccine mandates have been implemented are associated with a 2.2% decrease in COVID-19-related deaths. Contrarily, the association between mask mandates and COVID-19-related deaths is statistically significant but unexpectedly positive. This is potentially because mask mandates are implemented during periods of high COVID-19 cases giving rise to a positive correlation between mask mandates and death rates. Additionally, the study reveals a significant positive association between population sizes and COVID-19-related deaths, emphasizing the effect of population density on virus transmission. COVID-19-related deaths also exhibit an expected negative relationship with state-level Gross Domestic Product (GDP) β more affluent states are equipped with the resources to minimize the health effect of diseases.
The study has several limitations. As it is based on observational data, potential endogeneity issues may arise, stressing the need to interpret the results as correlations, not causations. Moreover, aggregating data at the quarterly level gets rid of important insights that are visible with higher frequency data.
The remainder of the study is structured as follows. Section 2 offers a review of the related literature. Section 3 discusses the data, descriptive statistics, and the econometric model. Section 4 presents and discusses the findings, while Section 5 concludes.
Related Literature
The COVID-19 pandemic prompted widespread public health interventions, with governments worldwide implementing various measures to contain the spread of the virus. Among these interventions, mask mandates and vaccine requirements were key strategies to mitigate transmission. Researchers have debated the effectiveness of these interventions in containing the virus, raising questions about whether they were motivated primarily by political agendas rather than well-documented research findings. Consequently, this issue has garnered attention in numerous research studies.
Among earlier studies on this topic, Chu, et al. (2020) conducted a comprehensive meta-analysis focusing on the effects of physical distancing, face masks, and eye protection on virus transmissions [10]. Utilizing data from WHO-specific and COVID-19 specific sources, they performed frequentist and Bayesian meta-analyses, including random-effects meta-regressions. Analyzing 172 observational studies across multiple countries, they discovered that maintaining a physical distance of 1 meter or more lowers virus transmission, with protection increasing as distance increases. Additionally, face masks and eye protection were found to reduce the risk of infections. Liang, et al. (2020) obtained similar results in their systematic review and meta-analysis [2].
Asadi, et al. (2020) investigated whether mask-wearers emit particles into the surrounding air [2,3]. Their study, measuring micron-scale particle emission by individuals wearing different types of masks during expiratory activities, revealed that surgical masks and KN95 respirators significantly decreased outward emission rates during speaking and coughing compared to individuals without masks. Homemade cotton-fabric masks’ efficacy was inconclusive due to confounding factors, necessitating further research. Howard, et al. (2021) developed an analytical framework, establishing that mask-wearing reduces transmission of infected respiratory particles, particularly when public compliance is high [4].
Guerra & Guerra (2021) studied mask mandate efficacy on a state-level basis, analyzing COVID-19 case growth and mask use across the United States [5]. Their results suggested insignificant differences in case growth between mandate and non-mandate states, indicating no clear link between mask mandates/use and slower state-level COVID-19 spread. Motallebi, et al. (2022) focused on face-covering mandates’ effect on COVID-19 mortality rates in 44 countries [6]. Their cohort study, controlling for various confounders, revealed a significant mortality reduction in countries with face mask mandates.
Ahmad, et al. (2021) explored the effectiveness of face masks in containing SARS-CoV-2 spread, raising concerns about potential respiratory issues for individuals with underlying conditions [7]. Several other studies also documented the effectiveness of facemasks [10-15], suggesting an overall consensus on the effectiveness of mask mandates in containing virus spread.
Regarding immunities and death rates, controversies arise from concerns about long-term side effects, institutional mandates’ strength, and ethical issues surrounding compulsory mandates, research, testing, informed consent, and access disparities [16-18]. Gazi,t et al. (2021) investigated natural immunity versus vaccine-induced immunity, concluding that natural immunity is stronger and longer-lasting for COVID-19 infection, hospitalization, and symptomatic disease [8]. Acton, et al. (2022) demonstrated that vaccine mandates at colleges reduced COVID-19 cases and deaths in the fall 2021 semester [9].
The current study contributes to this literature by utilizing quarterly data on all fifty states of the United States and the District of Columbia over the period 2020-2021 and estimating a fixed effects multiple linear regression model. This approach, zooming in on vaccine and mask mandates, along with demographic, social, and clinical covariates, controls for state-specific, time invariant fixed effects and assesses the significant association between different states’ mandates and death rates.
Data and Econometric Model
Data and Summary Statistics
The dataset is structured as a panel dataset with 408 observations for all fifty states and the District of Columbia, for all four quarters of 2020 and 2021. Each quarter is interpreted as a typical calendar year (Q1/Q2/Q3/Q4). Our data sources are presented in Table 1.
Definition Of the Study Variables
COVID-19-Related Data: COVID-19-related information was gathered from various sources. The vaccine mandate data was obtained from the Kaiser Family Foundation (KFF), which categorized each state based on the presence or absence of a vaccine mandate, along with the specific timeframe during which the mandate was active. This data was utilized to assess the effectiveness of vaccine mandates in comparison to states without such mandates. Additionally, the mask requirement data was extracted from Wikipedia, where each state was segmented by the start and end dates of the mask mandate. These dates were substantiated with references to external sources, including articles and government websites. Both the vaccine mandate and mask requirement variables are presented as binary variables, where (=1) signifies the presence of the mandate during that quarter, and (=0) indicates the absence of the mandate during that period.
Macroeconomic Data: Macroeconomic data was sourced from the U.S. Census Bureau, St. Louis FRED, and the Centers for Medicare & Medicaid Services (CMS). Quarterly GDP figures were extracted for each state from the St. Louis FRED database, providing insights into economic activity and to see if individuals were in close contact with one another. Total unemployment serves as another indicator of financial and economic health, with an uptick suggesting a decline in economic wellbeing during the specified period.
Medicare eligibility data, acquired from CMS, was utilized to establish a new metric termed ‘atrisk population.’ This metric was obtained by dividing the number of Medicare eligible individuals in a state by the total population of the state, offering a proportion that represents elderly individuals. This metric aids in assessing the impact of additional elderly individuals, who have a heightened mortality risk compared to under-sixty-five individuals, on COVID-19 deaths.
The population variable, sourced from the U.S. Census Bureau and aggregated to a quarter, contributes to the analysis of population density within each state. Additionally, it also played a role in the creation of the ‘at-risk population’ variable, helping gauge the proportion of elderly individuals relative to the total population in each state.
Mortality Data: Mortality data was gathered from the Centers for Disease Control and Prevention (CDC), presented on a monthly basis for each state spanning from 2020 to 2022. For the analysis, we aggregated the data for each state to a quarter by calculating the average over three-month intervals. The deaths were further categorized into those attributed to COVID-19 and those associated with all causes. Leveraging these variables, we computed the proportion of deaths specifically attributed to COVID-19 relative to the total number of deaths.
Descriptive Statistics
The average COVID-related death rate over the eight quarters in the sample for all fifty states and the District of Columbia is 693 people, whereas the average total death rate is 5,633 people. Both death rate measures exhibit significant standard deviations, indicating substantial cross-sectional and time variations across state-years. Notably, California in the first quarter of 2021 (with 10,036 deaths) and Utah in the first quarter of 2020 (0 deaths) had the highest and lowest COVID deaths respectively, while California in the first quarter of 2021 (35,003 deaths) and Alaska in the second quarter of 2020 (371 deaths) had the highest and lowest total deaths over the sample period. Approximately 10.6% of total deaths over the entire sample are attributed to COVID-19.
The average unemployment rate, standing at 6%, reflects the challenges the labor market faced in the recovery from the pandemic. Throughout the sample, 12% of state-years had a vaccine mandate, while approximately 43% had a mask mandate. The average state-quarter GDP for the sample period was $427,569 billion, with California boasting the largest economy in the last quarter of 2021 (over $3.5 trillion) and Vermont’s economy in the second quarter of 2020 ($30.6 billion) being the smallest. California also holds the highest population in first quarter of 2020 (about 39.5 million people), while Wyoming in the same quarter has the lowest population (577 thousand people).
An average of 1.2 million people were covered by Medicare, with California having the largest number benefiting from Medicare coverage, and the District of Columbia having the smallest. Our measure of ‘at-risk population,’ defined as the ratio of people eligible for Medicare coverage to the total state population, has an average value of 19.3%, meaning roughly 1 out of 5 individuals had an elevated mortality risk during the sample period. Maine tops the list in the last quarter of 2021, while Utah was at the bottom in the first quarter of 2020 (Table 2).
Econometric Model and Method of Estimation
The econometric model adopted in this study is a multiple linear regression model designed to explain COVID-19-related
deaths. Given that we are utilizing state-level quarterly panel data from 2020 and 2021, the formulation of the econometric model is as follows:
Yit=β0+β1x1,it+β2x2,it+...+βkxk,it+αi+uit
Where the subscript π denotes states and π‘ denotes quarters spanning from the first quarter of 2020 to the last quarter of 2021. The term πΌπ captures time-invariant fixed effects for each state, while π’ππ‘ represents the error term.
The dependent variable in our model (πππ‘) is the ratio of COVID- 19-related deaths to total deaths in each state every quarter. The explanatory variables (the π₯ππ‘’s) incorporated into our econometric model aim to encompass macroeconomic, demographic, and health factors for each state. These variables include the natural logarithm of the state’s quarterly GDP, the unemployment rate, and the natural logarithm of the state’s population. Additionally, our regression equation includes a measure of the at-risk population.
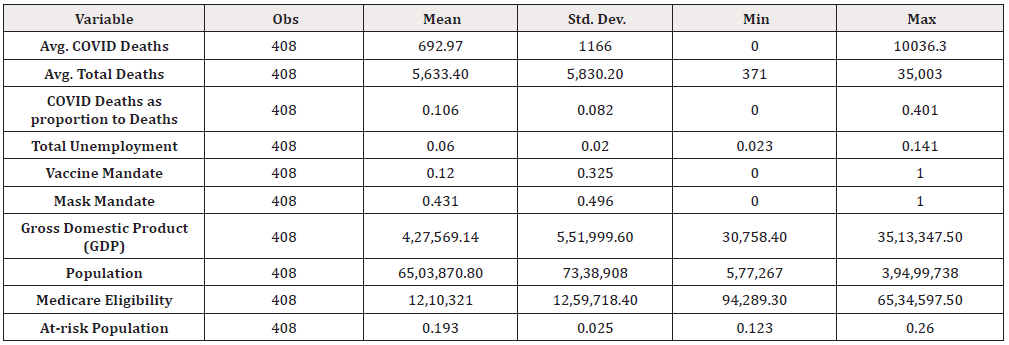
Table 2: Summary Statistics.
Note*: Source: Own computations, data from Census Bureau, St. Louis FRED, and the Centers for Medicare & Medicaid Services (CMS), Centers for Disease Control and Prevention (CDC).
Therefore, the regression equation we estimate is given by
π·πππ‘βπ ππ‘ππ ππ‘= π½0 + π½1πππππππππππππ‘ππ ππ‘+ π½2πππ πππππππ‘ππ ππ‘
+ π½3ππππππππ¦ππππ‘π ππ‘πππ‘+ π½4log (πΊπ·π)ππ‘+ π½5log (ππππ’πππ‘πππ) ππ‘+ π½6π΄π‘π ππ πππππ’πππ‘πππππ‘+ πΌπ+ π’ππ‘
πΌπ and π’ππ‘ are the fixed effects and the error term, respectively, as before.
We estimate our regression using both pooled Ordinary Least Squares (OLS) and fixed-effects panel data techniques. Pooled OLS treats the 408 observations as distinct entities, disregarding the panel structure of the data. Given that each state and the District of Columbia in our sample have 8 observations (four quarters for 2020 and 2021 each), the pooled OLS estimate results in biased coefficient estimates due to state-specific variables that are related to the geographic location of the state, the state’s laws, and so on. etc. Therefore, we present it for comparison purposes only.
Our primary inferences are drawn from the fixed-effects regression. This technique acknowledges the panel structure of the data and eliminates individual fixed effects through data transformations. Consequently, state-specific factors do not introduce bias to the fixed-effects results.
Results and Discussion
We present the regression results in Table 3 below. As mentioned previously, our primary interpretations and inferences will rely on the fixed effects regression results. However, it is noteworthy that several variables that are estimated to be insignificant with the pooled OLS technique attain significance when accounting for the panel structure of the data. Additionally, the R-squared from the fixed effects model is 28.5%, markedly higher than that of the pooled OLS. This suggests that the covariates included in the regression equation collectively account for 28.5% of the variation in COVID-19 related deaths as a ratio of total deaths with the fixed effects regression (Table 3).

Table 3: Regression Results.
Note*: Robust standard errors in parentheses *** p<0.01, ** p<0.05, * p<0.1
The estimated coefficient for vaccine mandates is indeed negative and statistically significant at the 10% level. Holding all else constant, this coefficient implies that states and periods with implemented vaccine mandates are associated with a significant 2.2% decrease in COVID-19related deaths relative to total deaths, compared to states and periods without such mandates.
While the negative sign aligns with our expectations, significance only at the 10% level and seemingly modest magnitude warrant further elaboration. The impact of vaccine mandates extends beyond individual immunization; it contributes significantly to herd immunity, amplifying the overall effectiveness of the vaccine in curbing the spread of viruses. Thus, the observed modest magnitude and lower significance may be attributed in part to the constraints imposed by our small sample.
Furthermore, vaccination rates tend to increase over time, while our dummy variable remains fixed at 1 from the first quarter of its implementation. This static nature may not fully capture the evolving impact of rising vaccination rates. Incorporating the actual number of vaccinated individuals into the regression analysis could yield more robust and meaningful results, potentially offering a better understanding of the role played by vaccine mandates in mitigating COVID-19 cases and deaths.
The association between mask mandates and COVID-19-related deaths is statistically highly significant. However, the unexpected positive sign of the coefficient contradicts intuition. Intuitively, one would expect that the implementation of mask mandates, holding all else constant, should result in fewer deaths as it helps contain the spread of the virus. The coefficient obtained, however, is positive, suggesting that periods with mask mandates are associated with 4.2% higher COVID-19-related deaths compared to periods without mask mandates.
While the counterintuitive sign might raise eyebrows, a compelling explanation supports this finding. Mask mandates were likely implemented during periods of high COVID-19 cases. The positive association between mask mandates and COVID-19-related deaths captures this nuanced relationship – mask mandates are potentially a response to high COVID-19-related deaths, giving rise to a positive association between mask mandates and COVID-19 deaths. This aligns with the key limitation we highlighted in the introduction of our study – the results we report signify correlation rather than causation.
We also found an intuitive result related to our measure of the at-risk population. The coefficient for the at-risk population is 0.181, signifying that a 1% increase in the proportion of at-risk population is associated with an 18.1% increase in COVID- 19-related mortality as a proportion of total deaths, keeping all other factors constant. This finding aligns with our expectations, considering that older populations, constituting the at-risk segment, were significantly more affected than their younger counterparts during the pandemic.
The additional findings we found not only align with intuition but also confirm our initial expectations. Firstly, we observed that unemployment rates are not significantly linked to death rates. This could be attributed to the dual impact of unemployment on COVID-19 cases, where the potential negative effect of unemployment on the standard of living and health conditions may be offset by the positive health aspects of reduced social contacts due to people staying home. Secondly, in terms of Gross Domestic Product (GDP), our results indicate that higher GDP, which translates into the existence of more resources to combat the virus’s spread and provide adequate medical care to individuals upon contracting the virus, is significantly associated with lower COVID-19-related deaths. Finally, when considering the effect of population size, a higher population size, all else being equal, correlates with increased population density. This higher density makes social distancing more challenging and contributes to high spread of the virus.
Conclusion
This study examines the link between public health interventions and COVID-19-related mortality rates in the United States. Utilizing state-level quarterly data spanning 2020-2021, we conduct a fixed effects regression, examining the impact of vaccine and mask mandates alongside demographic, health, and economic variables on death rates. Our analysis reveals an expected decrease in death rates associated with vaccine mandates, while unexpectedly, mask mandates exhibit a positive correlation with mortality rates. This counterintuitive finding may stem from the fact that these policy interventions are a response to high death rates, establishing a positive correlation between mask mandates and high death rates. Additionally, we find a positive relationship between population size and COVID-19-related deaths and a negative correlation between the level of GDP and COVID-19-related death rates. As an area for future research, a study that utilizes higher frequency data, along with the actual number of vaccinations and a proxy for actual usage of facial coverings, can offer additional insights into our findings.
Acknowledgments
The authors would like to thank participants of the research seminar at Lake Forest College.
Conflicts of Interest
None.
References
- Chu DK, Akl EA, Duda S, Solo K, Yaacoub S, Schünemann et al. (2020) Physical distancing, face masks, and eye protection to prevent person-to-person transmission of SARS-CoV-2 and COVID-19: A systematic review and meta-analysis. The Lancet 395(10242): 1973-1987.
- Liang M, Gao L, Cheng C, Zhou Q, Uy JP, et al. (2020) Efficacy of face mask in preventing respiratory virus transmission: A systematic review and meta-analysis. Travel Med Infect Dis 36: 101751.
- Asadi S, Capp CD, Barreda S, Wexler AS, Bouvier NM, et al. (2020). Efficacy of masks and face coverings in controlling outward aerosol particle emission from expiratory activities. Scientific Reports 10(1): 1-13.
- Howard J, Huang A, Li Z, Tufekci Z, Zdimal V, et al. (2021) An evidence review of face masks against COVID-19. Proc Natl Acad U S A 118(4): 2014564118.
- Guerra DD, Guerra DJ (2021) Mask mandate and use efficacy for COVID-19 containment in US States [Preprint]. Epidemiology.
- Motallebi S, Cheung RCY, Mohit B, Shahabi S, Alishahi Tabriz A, et al. (2022) Modeling COVID-19 Mortality Across 44 Countries: Face Covering May Reduce Deaths. Am J Prev Med 62(4): 483-491.
- Ahmad MF, Wahab S, Ahmad FA, Alam MI, Ather H, et al. (2021) A novel perspective approach to explore pros and cons of face mask in prevention the spread of SARS-CoV-2 and other pathogens. Saudi Pharmaceutical Journal 29(2): 121-133.
- Gazit S, Shlezinger R, Perez G, Lotan R, Peretz A, et al. (2021) Comparing SARS-CoV-2 natural immunity to vaccineinduced immunity: Reinfections versus breakthrough infections [Preprint]. Infectious Diseases (except HIV/AIDS).
- Acton RK, Cao W, Cook EE, Imberman SA, Lovenheim MF (2022) The effect of vaccine mandates on disease spread: Evidence from college COVID-19 mandates. National Bureau of Economic Research.
- Chou R, Dana T, Jungbauer R, Weeks C, McDonagh MS (2020) Masks for prevention of respiratory virus infections, including SARS-CoV-2, in health care and community settings: A living rapid review. Ann Inter Med 173(7): 542-555.
- Joo H, Miller GF, Sunshine G, Gakh M, Pike J, et al. (2021) Decline in COVID-19 hospitalization growth rates associated with statewide mask mandates—10 states, March–October 2020. Morbidity and Mortality Weekly Report 70(6): 212-216.
- Li L, Li, B, Liu SH, Ji J, Li Y (2021) Evaluating the impact of New York’s executive order on face mask use on COVID-19 cases and mortality: A comparative interrupted times series study. J Gen Intern Med 36(4): 985-989.
- Liao M, Liu H, Wang X, Hu X, Huang Y, et al. (2021) A technical review of face mask wearing in preventing respiratory COVID-19 transmission. Curr Opin Colloid Interface Sci 52: 101417.
- Lyu W, Wehby GL (2020). Community Use of Face Masks And COVID-19: Evidence from A Natural Experiment of State Mandates In The US: Health Aff 39(8): 1419-1425.
- Shen M, Zu J, Fairley CK, Pagán JA, Ferket B, et al. (2021) Effects of New York’s executive order on face mask use on COVID-19 infections and mortality: A modeling study. J Urban Health 98(2): 197-204.
- Amin ANE, Parra MT, Kim Farley R, Fielding JE (2012) Ethical issues concerning vaccination requirements. Public Health Reviews 34: 1-20.
- Isaacs D, Kilham H, Leask J, Tobin B (2009) Ethical issues in immunisation. Vaccine 27(5): 615-618.
- Lantos JD, Jackson MA, Opel DJ, Marcuse EK, Myers AL, et al. (2010) Controversies in vaccine mandates. Curr Probl Pediatr Adolesc Health Care 40(3): 38-58.