Research Article
Creative Commons, CC-BY
COVID-19 Epidemic Models: A Study from Georgia State in the USA
*Corresponding author: Hafiz Khan, Julia Jones Matthews Department of Public Health, Texas Tech University Health Sciences Centre, Lubbock, TX 79430, USA
Received: September 17, 2020; Published: October 23, 2020
DOI: 10.34297/AJBSR.2020.10.001518
Abstract
The purpose of this study was to identify gender-specific differences and best-fit coronavirus (covid-19) model for the infected people of Georgia. Statistical methods chi-squared, ANOVA, logistic regression, Poisson and negative binomial regression models were utilized to analyze Covid-19 data, which were obtained from the Georgia Department of Public Health. The difference among the mean ages of deaths for overall underlying conditions (P = 0.0248) and with ‘no’ and ‘unknown’ medical conditions (P = 0.0196) were found to be significant. The covariates regions, minimum age, maximum age, and average age were found to have a significant effect (P < 0.0001). The negative binomial regression model exhibited a best-fit model in building a death curve compared to Poisson regression model obtained by the GLM method. The findings will help to determine genderspecific future virus models for effective interventions, and they can be generalized to the population with geographic and racial/ethnic similarities.
Keywords: Coronavirus (COVID-19), Epidemic, Outbreak, Symptoms, Statistical methods
Introduction
Covid-19 is the novel coronavirus, which was first found in the city of Wuhan in China in November of 2019. The first source of interaction with the disease was found in a seafood market. Wuhan is known as an important hub in China and contains an international airport which may have rapidly increased the spread of the virus [1]. Similar to the Middle Eastern Respiratory Syndrome and the Severe Acute Respiratory Syndrome, the novel 2019 coronavirus had the same host, which was a bat. The bat was said to have infected a pangolin. Humans were then infected from the pangolin through the process of zoonosis [2]. Super-spreading can exponentially increase the number of individuals infected by the virus as the novel covid-19 has the potential to spread rapidly [3]. There are mainly two ways in which the disease can spread, one of them being the fecal-oral route. The fecal-oral route of transmission occurs when proper hygiene is not placed after an individual discards feces. Contamination of common bathroom areas can increase the spread of the disease through possible selfinoculation. Transmission of droplets from the mucous membranes can also transmit the virus [4]. Having good hygiene and washing hands after bathroom usage can help decrease the risk of infection.
Covid-19 has a high rate of spreading, causing roughly three people to become infected from each infected person. Symptoms of covid-19 include respiratory issues such as difficulty in breathing. Covid-19 is known to attack the alveoli and cause injury to the surfactants in the alveoli, causing alveolar collapse through the dramatic decrease in surface tension [5]. Surfactants are secreted into the type 2 cells in the alveoli and contain proteins and lipids. The majority of surfactants contain lipids [6]. The phospholipids present in the surfactants greatly improve the ability to reduce surface tension among the alveoli. Covid-19 can enter the body through the mucous membranes. Receptors in the body bind to the spikes on the virus, allowing it to enter into the cell. Once it enters the cell, the virus can replicate itself, creating more viral particles, which can inhibit the body.
Elderly individuals are at the highest risk of the virus with men infected more than women. Having other health diseases such as diabetes or cardiovascular disease increases the chance of contracting covid-19 significantly [7]. Having a strong immune system seems to be of utmost importance in fighting the covid-19. As mentioned in8, there is a 12.15% higher percent chance in contracting covid-19 among individuals who are 80 years of age or older in comparison to middle-aged individuals in the age group of 50 years or older who have a 1.25% chance of getting covid-19. This number continues to decrease, as age becomes less. Having pre-existing health conditions and being immunocompromised can increase the likelihood of getting covid-19 [8]. Older people with other medical conditions such as asthma, diabetes, or heart disease may be more vulnerable to becoming severely ill [9].
There are numerous ways to decrease the rate of the spread of the virus. One effective way is to slowly decrease and ultimately cancel mass gatherings. Studies have shown that a prominent way of virus transmission can occur through mass gatherings where individuals are close together in an area, whether it is an indoor or outdoor event. Efforts to prevent high transmission of the virus resulted in the closure of sporting events, music events such as concerts, and small group gatherings, all of which are highly discouraged. Temporarily closing down religious places of worship such as churches have been implemented to help prevent the spread of the virus [10].
As mentioned by Liu et al. [7], lung infections are a prominent symptom of covid-197. Common symptoms of the novel coronavirus are flu-like, although there are individuals who have asymptomatic features of the virus and do not show easily recognizable symptoms. According to the World Health Organization, symptoms of covid-19 are nasal congestion, dry cough, fever, diarrhea, shortness of breath, runny nose, among other symptoms [11]. The usual time of symptoms occurs within two to fourteen days after having contact with the virus [12]. There is currently no vaccine to prevent covid-19 and the best way to prevent illness is to avoid being exposed to this virus [13].
In the rapid progress of covid-19 pandemic, a huge amount of data has been collected from thousands of subjects, which raises concerns on how one can analyse such data and talk the scientific language to the public. Statistical and computational techniques are very useful at the cutting edge to understand such data and to make scientific conclusions. There is an urgency to utilize statistical methods on covid-19 epidemic data to know the patterns of the disease progression, intervention, and prevention. Though at the initial stage there is no direct preventive method exist but recorded data can lead the knowledge of variability and predictability of this disease due to some demographics, and thus can apply interventions to reduce its impact. Covid-19 data are collected by many agencies including hospitals, clinics, public health labs, health organizations, etc. The immediate release of such data for public access will accelerate the research to find intervention such as a vaccine or preventive medicine to stop the spread of this pandemic. Fortunately, we obtained limited publicly accessible demographic data from the Georgia Department of Public Health (GDPH) [14]. Using this data, we investigated the appropriate statistical methods and algorithms to visualize disease occurrences/confirmed cases, recovery cases/alive, and total deaths.
This study aimed to explore whether gender-specific differences exist in GDPH covid-19 data and to obtain the best-fit statistical model for death occurrences. The findings of this study will assist to (i) identify infected confirmed cases among males and females with covid-19 through descriptive analysis of accessible sociodemographic variables, (ii) conduct test of hypothesis for ages of deaths and multiple comparison test against covariates such as gender and underlying medical conditions, (iii) perform a Pearson chi-square test to check the independence of gender and underlying medical conditions, (iv) carry out a logistic regression method with certain covariates for individual-level data, and (v) utilize a generalized linear model to build a best-fit model using aggregate level data on the number of deaths.
Methods
Data source/variables and study population
The data of 156 counties was collected from the GDPH [14], of which 81 counties had deaths. Covid-19 individual-level data was not made available for public viewing except some aggregate level. However, the GDPH had released de-identified covid-19 data both for aggregate as well as individual levels. Variables such as age, gender, cases, alive, deaths, counties, and underlying medical conditions (yes, no, unknown) were reported to the public. The number of confirmed cases, hospitalizations, and deaths were 12159, 2479, and 428, respectively.
The age variable was continuous and grouped into three subgroups such as age1: 20-40 years, age2: 41-60 years, and age3: 61 years and above. The total number of confirmed cases within 156 counties in aggregate levels were grouped into four subgroups (0< region1<100; 100≤ region2<200; 200≤region3<300; 300≤ region4) to find the distributional differences of covid-19 occurrences among the subgroups. Logistic regression was used to illustrate the odds of an event (deaths/alive), given some demographic covariates. Poisson and negative binomial regression models [15,16] were used to find the best-fit death model based on aggregate level data.
Sample size calculations
The sample size was calculated using G*Power software package [17]. It was determined that 159 participants, 53 in each independent group, was sufficient to compare mean differences of continuous measurements when running a one-way analysis of variance (ANOVA) test. It was estimated that a total of 108 subjects would be sufficient to detect a statistically significant relationship for two discrete variables when running a Pearson’s chi-squared test for a 2 x 3 contingency table.
Data (n1 = 12,159 total confirmed cases, n2 = 2,479 hospitalized, and n3 = 428 deaths) from 156 counties in which deaths were observed from 81 counties. It was calculated that 428 subjects would be large enough for logistic regression to compare the gender-specific differences. All the calculations were based on alpha (α) = 0.05, power = 80%, and a two-sided testing procedure.
Statistical analysis
The R-GUI software package [18] was used to perform analysis. Since the dependent variable was categorical (covid-19 positive and negative), a logistic regression method was performed to calculate odds ratios and its 95% confidence intervals to determine the association between risk factors due to the occurrence of covid-19. For the count data, the appropriate regression models are Poisson regression and negative binomial regression. Generalized Linear Models (GLM) can incorporate binary data, count data, and skewed data to model response variable as a function of covariates through assumptions on exponential family such as binomial, Poison, negative binomial, and others. The GLM was used by Khan et al. [19], to obtain inferences about parameters under three sampling plans [19]. The Poisson and negative binomial regression models were used for the analysis of covid-19 deaths data at aggregate level. The ages of deaths for both males and females were grouped with the underlying medical conditions. ANOVA was performed on each gender separately, and also combining both genders age with underlying medical conditions to detect whether the mean ages of deaths is significant. A Pearson chi-square test was used to detect if there is a significant relationship between gender and underlying medical conditions.
Results
This study investigated gender differences associated with covid-19 among patients living in 156 counties where 81 counties had deaths in Georgia. [Table 1] contains the summary results of logistic regression for the binary outcome and for sociodemographic variables age, age groups, minimum, maximum, average age, gender, underlying medical conditions (MC0, MC1, and MC2), counties, regions, deaths, and alive. The MC0 described no medication conditions usually referred to healthy people, MC1 was defined for those had underlying medical conditions, and MC2 was used for those medical conditions unknown.
[Table 1] depicts the summary statistics for the risk factors. No significant regression estimates were found for both males and females while running the logistic regression at the individuallevel data. Running a logistic regression for the deaths of male with covariates age, MC1, and MC2 when MC0 as the referent group; it was found that both MC1 and MC2 were protective but MC1 was observed very low likelihood of deaths at the individual level. The age variable was grouped into age1, age2, and age3 to detect whether any subgroup had a higher likelihood of covid-19 deaths. It was found that age2 had a very low likelihood of deaths considering age1 as a referent, and MC1 remained a higher chance of survival compared to MC2 with MC0 as a referent.
While partitioning aggregate level data into four regions (region1, region2, region3, region4) and using logistic regression we obtained region2 and region3 were significant for minimum and maximum age distributions. The region4 was found to be significant for the average age distribution at aggregate analysis.
[Table 2] reflects the ages of deaths for both males and females were aligned with their underlying medical conditions. Minimum, average, and maximum age for male and female deaths were: 29, 71.54, 98; and 22, 73.15, 100, respectively. The P-value for the equality of mean age of death for males and females was 0.2694, and hence we conclude that the average age of deaths for male and female was not differ significantly. Among 428 deaths, 68% had underlying medical conditions, 3% have no medical conditions and the remaining 29% have unknown conditions. The P-value of the Pearson’s chi-square test of independence for ‘underlying medical conditions’ and ‘gender’ was 0.2300, which indicates they were independent. We conducted three ANOVA tests, one for a male subgroup, one for a female subgroup, and one for overall.
ANOVA tests of male and female subgroups reveal that the average ages of deaths corresponding to each underlying medical condition were insignificant (P = 0.118 for males; P = 0.126 for females). However, for the overall group, ANOVA test for equality of several independent means test for each underlying condition was significant (P = 0.0248). Tukey’s multiple comparison test showed that a pair of means for underlying conditions ‘no’ and ‘unknown’ differ significantly (P = 0.0196), which ultimately caused the rejection of the overall ANOVA test.
The minimum, average, and maximum age were computed from the individual data corresponding to each county, which later merged with the county/aggregate level data to create three age variables in county level data. Poisson regression and a negative binomial regression model are appropriate when the dependent variable is count data, which was a number of deaths in our analysis. However, Poisson regression is more appropriate when the location parameter and dispersion parameter of the response variable are the same. Since our response variable was number of deaths, it had mean and variance as 5.28 and 102.23, respectively, which differ significantly, hence the Poisson regression model was not appropriate, because the fundamental assumption of using Poisson regression model was violated.
Negative binomial regression model is appropriate when the outcome variable is count, and the dispersion parameter is much higher than location parameter. Running the negative binomial regression model with the covariates (regions, minimum, maximum, average age) on the aggregate level data we obtained region2, region3, and region4 were highly significant considering region1 as the referent group. The odds ratios and confidence intervals divulged there were high likelihood of deaths found in region2, region3, and region4 compared with referent group region1 for aggregate level analysis. When running the logistic regression for deaths and covariates region2, region3, and region4 at aggregate level, it was found that the odds ratios were below 1.0, which indicates that the odds of coronavirus exposure among patients were lower and protective against the disease. The region4 was found to be more protective against the disease than region2 and region3 [Tables 1 & 2].

Table 1: Summary Results for Estimated Coefficients Using Logistic Regression/Generalized Linear Model.
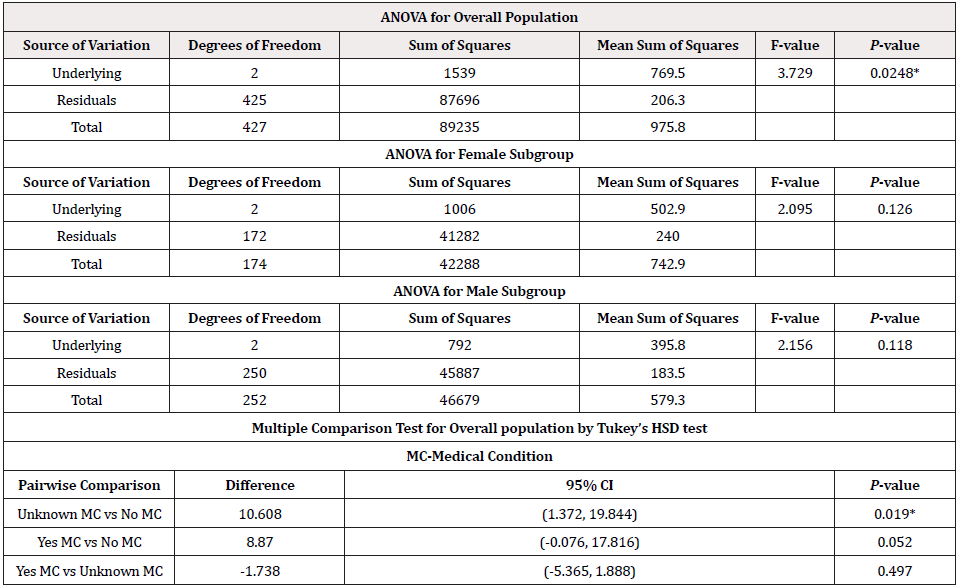
Table 2: Summary Results for ANOVA and Multiple Comparison Tests at Individual Level.
Note: CI=confidence interval MC=medical condition HSD=honest significance difference * P<0.05
[Figure 1] illustrates a comparison among gender-specific age of deaths, underlying medical conditions, and overall age of deaths at individual level. A histogram displays the deaths of females started at age 20 and had a peak at age 80-90 with a good proportion of deaths. Deaths of males begun at age 30 and had a peak at age 70-80, had a little drop at age 80-90, and after aged 90 there was a huge drop. The histogram of ages for overall deaths at individual level reached a peak between 80-90, which was almost same as 60-70. The distribution of ages of deaths was skewed to the left, which were confirmed by the long-left tail from histograms as well as outliers from the box plots. Bar charts exhibit that males had a higher death than females. There were a moderate number of males and females with approximately equal proportion to have unknown underlying medical conditions. It was observed that the male population had slightly higher proportions of having medical conditions and no medical condition compared to their female counterpart. It was evident that covid-19 was disproportionately rising higher risk of killing males compared to females.
[Figure 2] depicts a visual representation of boxplots of number of cases and deaths for four regions at aggregate level. The boxplots and histograms were displayed for the maximum, minimum, and average age of deaths at county level. The histograms show the highest number of deaths occurred in age intervals 60-70, 80-85, and 70-80 for minimum, maximum and average age, and outliers were noticed in boxplots.
The boxplots for number of cases and deaths for each of the four regions clearly showed an increasing trend of occurrences due to coronavirus. The region4 contributed the highest number of cases, recoveries, deaths, and hospitalizations. It was independently verified that these trends of occurrences were consistent with the recoveries and hospitalizations. The stack bar chart in [Figure 2] reflects one bar had a high altitude but no death or few deaths. This bar was for those patients who were unable to report the county, they lived. The bar chart indicated that the bars that have high altitude also had high covid-19 cases and deaths. Deaths and alive were demarcated by red and white colour in the stack bar chart. The higher proportion of red was visualized for a higher death in the county.
Discussion and Future Directions
The covid-19 pandemic is a global public health threat and is the greatest challenge we have faced since World War Two [20]. It has the potential to create devastating social, economic, and political crises that leave us deep scars. The World Health Organization defines public health surveillance as “the continuous, systematic collection, analysis, and interpretation of health-related data needed for the planning, implementation, and evaluation of public health practice” and calls it the “bedrock of outbreak and epidemic response” [21]. As the covid-19 pandemic has progressed, the effectiveness of national efforts to combat the virus has hinged on the ability of governments to measure its spread and use that information to target their public health efforts.
Due to a lack of testing, monitoring and the resulting uncertainty about where covid-19 is spreading, national governments have deemed it necessary to put their entire populations on lockdown. The use of large public health data especially biological specimens will be extremely valuable to develop a biomarker for outcomes research, quality assurance, public health surveillance, and other beneficial purposes. When there will be a huge volume of recorded data available to the public, these analytical methods will carry over the benefit for researchers and public health practitioners to understand the nature of data and its rigorous statistical analysis.
Combining statistical methods and computer-based algorithms can play a significant part in generating statistical probabilistic models. The modelling approaches will provide us an understanding of existing covid-19 data and measure the risk of future pandemics in rural and urban communities.
The breakdown of the aggregate data into regions and using negative binomial regression on the number of deaths would be an appropriate method for future pandemic risk modelling direction to rural or urban areas. The findings of this study will expand to identify infected individuals for interventions and develop policy briefs for future pandemics.
Acknowledgements
The authors would like to thank the Georgia Department of Public Health for releasing limited data to public.
Human Subjects
No personal identifiable information was obtained.
Conflict of interests
The authors declare that they have no conflict of interests.
References
- Peeri NC, Shrestha N, Rahman MS, Zaki R, Tan Z, Bibi S, Haque U. The SARS, MERS and novel coronavirus (COVID-19) epidemics, the newest and biggest global health threats: what lessons have we learned? International Journal of Epidemiology. Available at: https://www.doi:10.1093/ije/dyaa033. Accessed March 2020
- Zhang T, Wu Q, Zhang, Z. Probable pangolin origin of SARS-CoV-2 associated with the COVID-19 outbreak. Current biology: CB. Available at: https://pubmed.ncbi.nlm.nih.gov/32197085/. Accessed March 2020.
- Lee A. Wuhan novel coronavirus (COVID-19): why global control is challenging? Public Health, 2020, 179. Available at: https://www.doi:10.1016/j.puhe.2020.02.001. Accessed March 2020.
- Hindson J. COVID-19: faecal–oral transmission? Nature Reviews Gastroenterology & Hepatology. Available at: https://www.doi:10.1038/s41575-020-0295-7. Accessed March 2020.
- COVID-19. Corona Virus: Epidemiology, Pathophysiology, Diagnostics. Available at: https://www.youtube.com/watch?v=PWzbArPgo-o. Accessed April 2020.
- Veldhuizen EJ, Haagsman HP. Role of pulmonary surfactant components in surface film formation and dynamics. Biochimica Et Biophysica Acta (BBA) - Biomembranes, 2020;1467(2): 255–270. Available at: https://www.doi:10.1016/s0005-2736(00)00256-x. Accessed March 2020.
- Liu K, Chen Y, Lin R, Han K. Clinical feature of COVID-19 in elderly patients: A comparison with young and middle-aged patients, Journal of Infection. Available at: https://www.doi.org/10.1016/j.jinf.2020.03.005. Accessed April 2020.
- What explains Covid-19's lethality for the elderly? Available at: https://www.statnews.com/2020/03/30/what-explains-coronavirus-lethality-for-elderly/. Accessed March 2020.
- Texas Department of State Health Services. Available at: https://www.dshs.texas.gov/coronavirus/. Accessed April 2020.
- Mccloskey B, Zumla A, Ippolito G, Blumberg L, Arbon P, Cicero A, … Borodina M. Mass gathering events and reducing further global spread of COVID-19: a political and public health dilemma. The Lancet. 2020;395(10230):1096–1099. Available at: https://www.doi: 10.1016/s0140-6736(20)30681-4. Accessed April 2020.
- World Health Organization. Q&A on coronaviruses (COVID-19) (n.d.). Available at: https://www.who.int/news-room/q-a-detail/q-a-coronaviruses#:~:text=symptoms. Accessed April 2020.
- Centers for Disease Control and Prevention. Symptoms of Coronavirus. Available at: https://www.cdc.gov/coronavirus/2019-ncov/symptoms-testing/symptoms.html. Accessed April 2020.
- Centers for Disease Control and Prevention. Coronavirus Disease 2019 (COVID-19). Available at: https://www.cdc.gov/coronavirus/2019-ncov/prevent-getting-sick/index.html, Accessed April 2020.
- Georgia Department of Public Health. Source of data. Available at: https://dph.georgia.gov/covid-19-daily-status-report. Accessed April 2020.
- Poisson Regression Model. Available at: https://www.statisticshowto.com/poisson-regression/. Accessed March 2020.
- Negative Binomial Regression Model. Available at: https://towardsdatascience.com/negative-binomial-regression-f99031bb25b4. Accessed March 2020.
- G∗Power version 3.1. Available at: https://gpower.software.informer. com/3.1/. Accessed on March 2020.
- R-GUI software version 3.6.3. The R project for statistical computing. Available at: https://www.r-project.org. Accessed March 2020.
- Khan HMR, Mende S, Rafiq A, Gabbidon K, Reddy PH. Methods needed to measure predictive accuracy: a study of diabetic patients. Biochim Biophys Acta (BBA) Mol Basis Dis 2017;1863(5):1046–1053.
- United Nations Development Programme. Available at: https://www.undp.org/content/undp/en/home/coronavirus.html. Accessed May 2020.
- Center for Global Development. Available at: https://www.cgdev.org/blog/covid-19-information-problems-and-digital-surveillance. Accessed May 2020.